Introduction: Why Technology is the Key Driver of Global Change
The 21st century has been marked by unprecedented technological advancements that have transformed every aspect of our lives. From the way we communicate to how we work, learn, and interact with the world, technology has been the backbone of innovation and progress. As we look ahead to the next decade, we find ourselves standing at the cusp of a new wave of transformative trends that promise to reshape industries, redefine economies, and revolutionize the human experience.
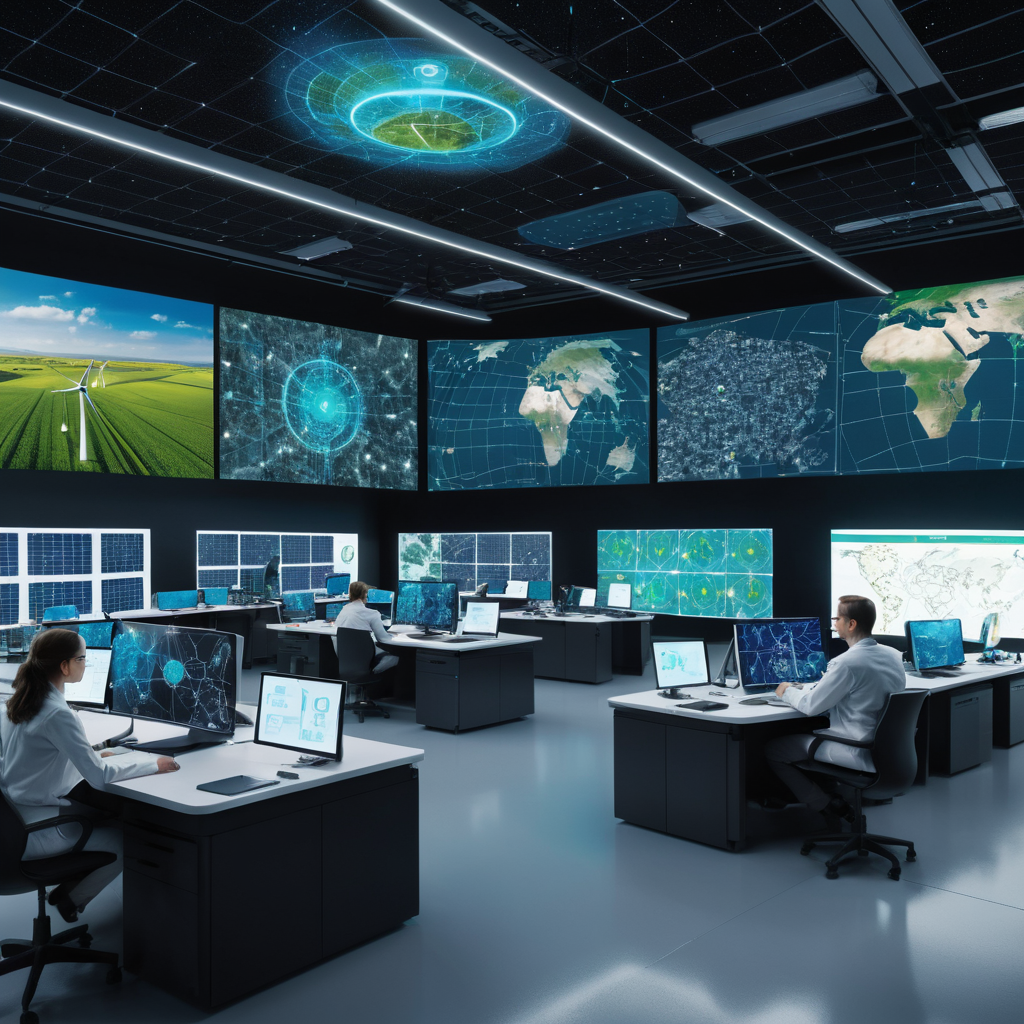
Table of Contents
In the past, technologies like the internet, smartphones, and renewable energy emerged as game-changers, altering the trajectory of humanity. The internet, for instance, turned the world into a global village, connecting billions and democratizing information access. Similarly, smartphones gave rise to the gig economy, social media, and on-demand services, forever changing the way we live and work. Renewable energy, particularly solar and wind power, began paving the way toward a sustainable future. These breakthroughs demonstrate how innovation can address critical global challenges and open new horizons of possibility.
Yet, the speed of change is accelerating. Experts estimate that technological advancements in the next 10 years will surpass those made in the last 50. This era of rapid evolution is driven by a confluence of emerging technologies, such as Artificial Intelligence (AI), Quantum Computing, and Green Tech, which are not just advancing in isolation but converging to create powerful synergies. These technologies will redefine the limits of what humanity can achieve, solving problems that were once thought impossible.
The Need to Understand Tech Trends
Understanding the emerging tech trends is not merely an academic exercise but a necessity for businesses, governments, and individuals. These trends have far-reaching implications:
- For Industries: Organizations that fail to adapt to new technologies risk becoming obsolete. For example, companies that ignored the rise of e-commerce, like traditional retail chains, faced massive closures as online marketplaces like Amazon grew exponentially.
- For Governments: Policymakers need to anticipate how these technologies will impact economies, labor markets, and societal structures. For instance, the rise of automation demands proactive planning for reskilling displaced workers.
- For Individuals: Staying informed about tech trends equips people with the knowledge to remain competitive in the job market, adopt smarter lifestyles, and make informed decisions about their future.
As history has shown, technology can be a double-edged sword. While it brings immense benefits, it also presents significant challenges. The internet revolutionized global communication, but it also gave rise to misinformation and cybercrime. Similarly, AI promises to make life more efficient but raises concerns about privacy, ethics, and job displacement. By understanding these trends, we can ensure that innovation is harnessed for the greater good.
The Five Tech Trends Poised to Reshape the Future
The blog focuses on five transformative technologies that will dominate the next decade:
- Artificial Intelligence (AI) and Machine Learning (ML): These are becoming the brainpower behind automation, decision-making, and problem-solving in industries like healthcare, finance, and education.
- Quantum Computing: A revolutionary computing model that could solve complex problems in seconds, from drug discovery to secure communications.
- Green and Sustainable Technologies: As climate change remains a pressing global issue, advancements in green tech are set to redefine energy, transportation, and agriculture.
- The Internet of Things (IoT) and Smart Cities: A future where everyday devices are interconnected to create smarter, more efficient urban living.
- Space Exploration and Colonization: The next frontier, where human innovation extends beyond Earth, opening new opportunities for exploration, resource extraction, and habitation.
Each of these trends carries immense potential to address critical challenges—whether it’s improving healthcare access, combating climate change, or ensuring global connectivity. However, they also come with ethical dilemmas, security risks, and societal disruptions that must be addressed.
A Vision of the Future
Imagine a world where:
- AI diagnoses diseases more accurately than any human doctor.
- Quantum computers solve complex climate models to reverse global warming.
- Green technologies power entire cities sustainably, with zero carbon emissions.
- IoT networks manage urban infrastructure seamlessly, reducing traffic congestion, improving energy efficiency, and enhancing public safety.
- Humanity establishes permanent colonies on the Moon and Mars, heralding a new era of space exploration.
This vision is not a far-off dream but a tangible reality within the next decade. However, achieving this future requires not just innovation but also collaboration across governments, industries, and individuals to ensure that technological progress benefits all of humanity.
Setting the Stage
As we delve into these five transformative trends, this blog will provide a detailed exploration of their current state, future potential, and the challenges that lie ahead. Drawing on insights from leading experts, notable books, and groundbreaking research, we aim to present a comprehensive guide to understanding the technologies shaping our tomorrow.
“Technology is best when it brings people together.” – Matt Mullenweg
With that in mind, let us explore the exciting tech trends that are poised to change the world in the next decade.
1. Artificial Intelligence (AI) and Machine Learning (ML): Transforming the Fabric of Society
Artificial Intelligence (AI) and Machine Learning (ML) are no longer just buzzwords; they are transformative technologies reshaping industries, societies, and the global economy. Over the past decade, AI has evolved from a specialized research field into a mainstream technology, influencing everything from healthcare and finance to education and entertainment. As we enter the next decade, the integration of AI and ML into everyday life will deepen, unlocking unprecedented possibilities while presenting new challenges.
AI refers to the simulation of human intelligence by machines capable of learning, reasoning, and problem-solving. ML, a subset of AI, focuses on algorithms that allow systems to learn from data and improve performance without explicit programming. Together, these technologies are redefining how we work, interact, and innovate.
A. Current Impact of AI and ML
Artificial Intelligence (AI) and Machine Learning (ML) have already transformed numerous industries and everyday life. These technologies are reshaping the way businesses operate, improving efficiency, and enabling innovation across sectors. The current impact of AI and ML spans various fields such as healthcare, finance, retail, and entertainment, driving growth and creating unprecedented opportunities. This section explores the significant contributions of AI and ML to different domains and their transformative role in modern society.
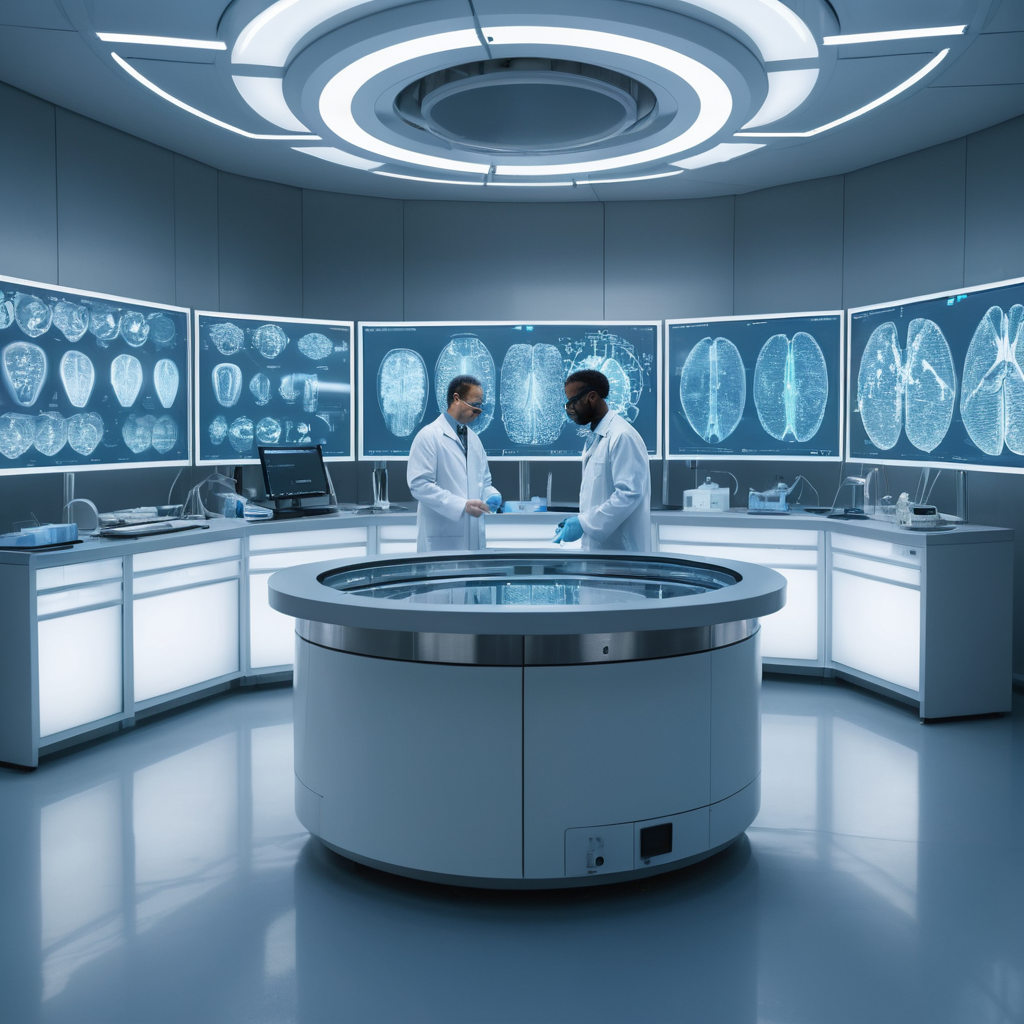
1. Healthcare: Transforming Diagnosis and Treatment
AI and ML are making healthcare more efficient, personalized, and accessible. These technologies are being used to diagnose diseases, predict patient outcomes, and recommend personalized treatments, revolutionizing the medical industry.
a) Enhanced Diagnostics
AI-powered diagnostic tools are enabling faster and more accurate detection of diseases. For example, AI algorithms analyze medical images, such as X-rays, MRIs, and CT scans, to identify anomalies with high precision. Studies have shown that AI models, such as those developed by Google Health, can detect breast cancer in mammograms with greater accuracy than human radiologists. Similarly, AI is being used to diagnose conditions like diabetic retinopathy, skin cancers, and cardiovascular diseases, often outperforming traditional diagnostic methods.
b) Personalized Medicine
Machine Learning algorithms are used to analyze patient data, including genetic information, to develop personalized treatment plans. By identifying unique genetic markers and understanding how patients respond to specific drugs, ML models enable healthcare providers to offer tailored therapies. For example, AI-driven platforms like IBM Watson Health assist in crafting individualized cancer treatments based on patient-specific data.
c) Predictive Healthcare
AI is also playing a critical role in predictive healthcare by analyzing patient history and lifestyle data to predict potential health risks. This allows for early intervention and preventive care, improving patient outcomes and reducing healthcare costs. Wearable devices like Fitbit and Apple Watch use AI to monitor vital signs and alert users to potential health issues, such as irregular heartbeats.
2. Finance: Driving Efficiency and Security
The financial sector has been one of the early adopters of AI and ML, leveraging these technologies to enhance operations, mitigate risks, and improve customer experiences.
a) Fraud Detection
AI and ML algorithms are widely used for fraud detection in financial transactions. These models analyze large volumes of data in real time, identifying unusual patterns or anomalies that could indicate fraudulent activity. For instance, credit card companies use AI to monitor transactions and flag suspicious activities, protecting users and businesses from financial losses.
b) Algorithmic Trading
In investment banking, AI-powered systems execute trades at lightning speeds based on data-driven insights. Algorithmic trading uses ML models to analyze market trends and predict stock movements, enabling traders to make more informed decisions. This has led to increased efficiency and profitability in financial markets.
c) Personalized Financial Services
AI-driven chatbots and virtual assistants, such as those used by banks like Bank of America’s Erica and Capital One’s Eno, provide personalized financial advice and customer support. These AI tools analyze user data to offer budgeting tips, track expenses, and recommend financial products tailored to individual needs.
3. Retail: Enhancing Customer Experiences
AI and ML are revolutionizing the retail industry by enabling businesses to understand customer preferences, optimize supply chains, and deliver personalized shopping experiences.
a) Recommendation Systems
E-commerce platforms like Amazon and Netflix utilize AI-driven recommendation systems to suggest products, movies, or shows based on user behavior. These systems analyze browsing history, purchase patterns, and preferences to create a personalized shopping or viewing experience, increasing customer satisfaction and sales.
b) Inventory Management
AI-powered tools help retailers optimize inventory levels by predicting demand and automating restocking processes. ML algorithms analyze historical sales data, market trends, and seasonality to ensure that businesses maintain optimal stock levels, reducing waste and storage costs.
c) Virtual Assistants and Chatbots
Retailers are increasingly using AI chatbots to assist customers with inquiries, provide product recommendations, and facilitate transactions. These virtual assistants operate 24/7, enhancing customer engagement and driving sales. For example, Sephora’s Virtual Artist leverages AI to allow users to try on makeup virtually before making a purchase.
4. Transportation: Revolutionizing Mobility
The transportation sector is undergoing a significant transformation due to the adoption of AI and ML technologies, which are improving safety, efficiency, and sustainability.
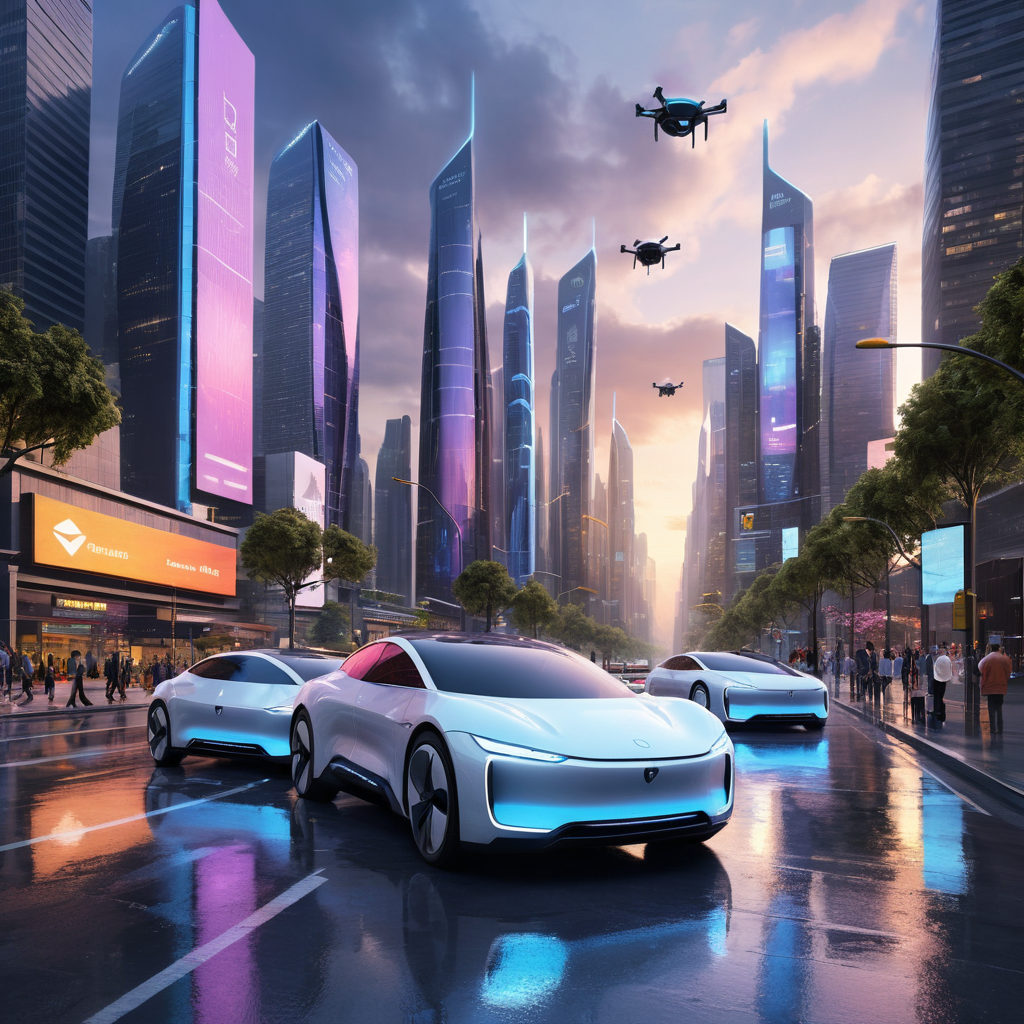
a) Autonomous Vehicles
Self-driving cars, powered by AI and ML, represent one of the most visible advancements in transportation. Companies like Tesla, Waymo, and Cruise are developing autonomous vehicles that use ML algorithms to analyze data from sensors, cameras, and radars, enabling them to navigate roads safely. These vehicles aim to reduce accidents caused by human error and improve mobility for people with disabilities.
b) Traffic Management
AI is also helping cities optimize traffic management systems. By analyzing data from traffic sensors and cameras, AI algorithms can predict congestion patterns and suggest real-time traffic adjustments, reducing delays and improving the flow of vehicles. Cities like Singapore and Los Angeles are already using AI-driven traffic control systems.
c) Predictive Maintenance
In logistics and aviation, AI is used for predictive maintenance, analyzing data from vehicles and machinery to identify potential issues before they become critical. This reduces downtime, enhances safety, and lowers operational costs.
5. Entertainment: Revolutionizing Content Creation
AI and ML are reshaping the entertainment industry by automating content creation, enhancing user experiences, and enabling innovative storytelling methods.
a) Content Recommendation
Streaming platforms like YouTube, Spotify, and Hulu use ML algorithms to recommend music, movies, and shows based on user preferences. These systems enhance user engagement and retention by providing highly personalized content suggestions.
b) AI-Generated Content
AI tools like OpenAI’s GPT and DeepAI are being used to create music, art, and even movie scripts. These systems use ML models to generate content that mimics human creativity, enabling faster production of high-quality entertainment.
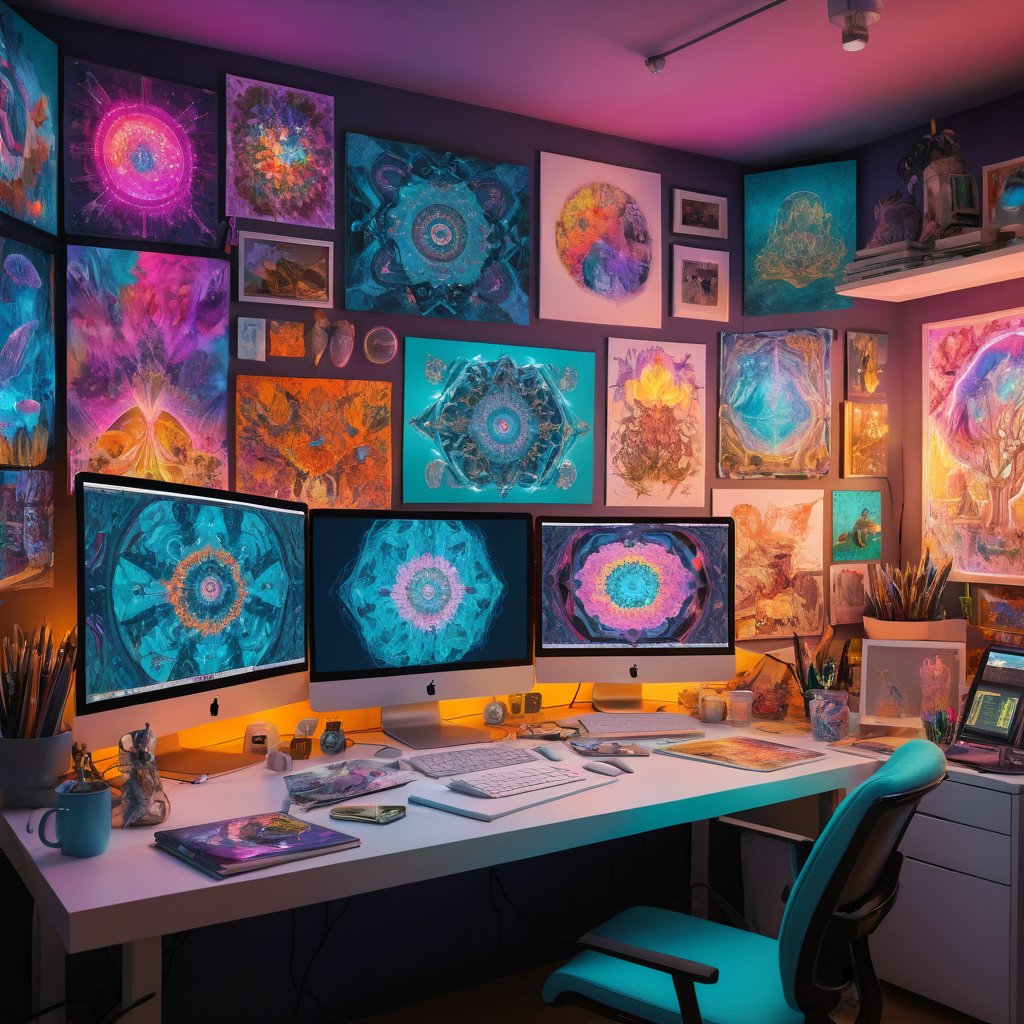
c) Virtual Reality (VR) and Augmented Reality (AR)
AI and ML are integral to the development of immersive VR and AR experiences. From gaming to virtual tours, these technologies analyze user interactions to create dynamic and engaging virtual environments.
The current impact of AI and ML is profound, touching nearly every aspect of modern life. From enhancing healthcare and securing financial transactions to revolutionizing retail and transforming transportation, these technologies have become indispensable tools for innovation and growth. As AI and ML continue to evolve, their influence will only expand, shaping a smarter, more efficient, and interconnected world.
B. Emerging AI Technologies
Artificial Intelligence (AI) is evolving rapidly, and emerging technologies are pushing the boundaries of what machines can achieve. These advancements promise to redefine industries, improve daily life, and address global challenges. In this section, we delve into the most promising emerging AI technologies, exploring their potential applications, benefits, and implications.
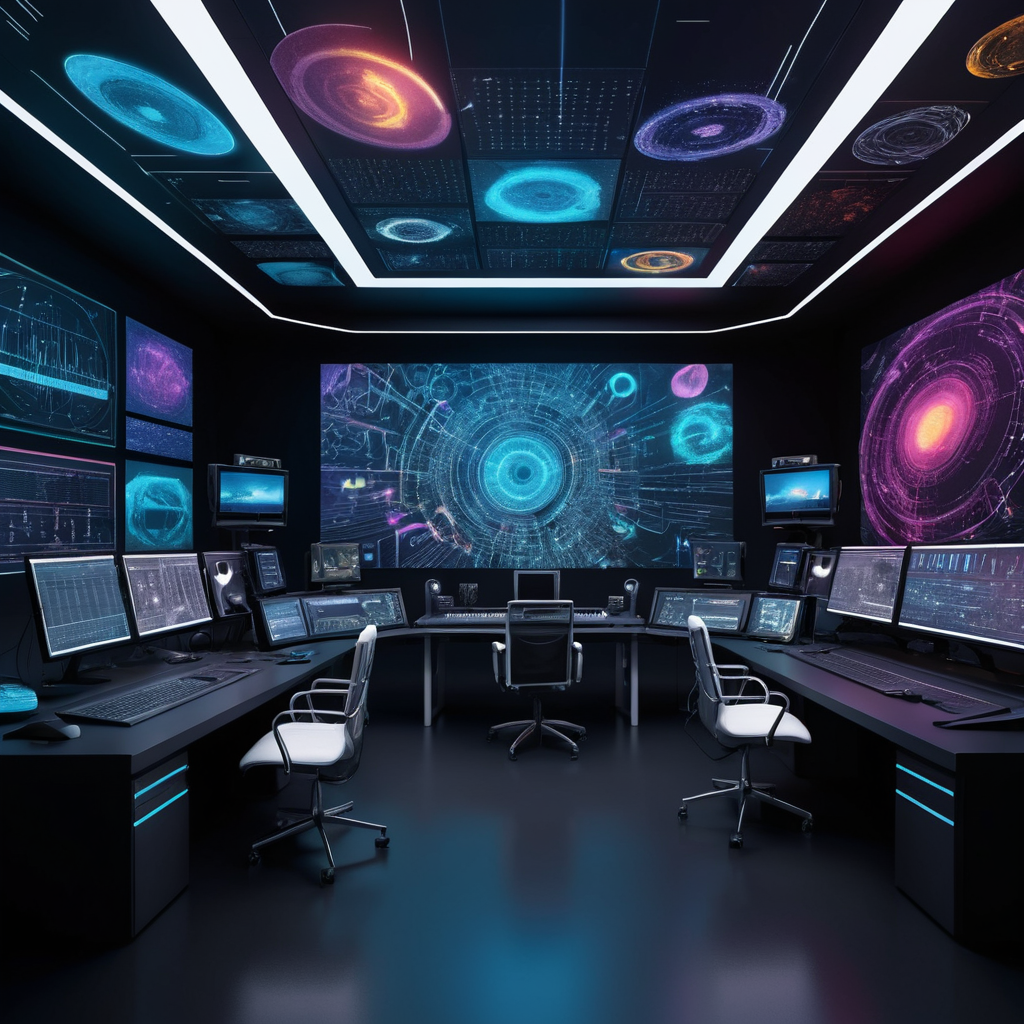
1. Generative AI: Creating Beyond Limits
Generative AI is a subset of artificial intelligence focused on creating new content. It uses sophisticated models to generate text, images, videos, and music, often indistinguishable from human-made creations.
a) Text Generation and Conversational AI
Models like OpenAI’s GPT series and Google’s Bard exemplify breakthroughs in generative text AI. These systems can craft essays, write code, and generate human-like conversations. Applications range from customer support chatbots to educational tools, revolutionizing how businesses and individuals interact with machines.
b) Image and Video Synthesis
Generative adversarial networks (GANs) are at the core of AI-generated images and videos. Tools like DALL-E and Stable Diffusion enable artists and creators to produce realistic visuals from textual descriptions. These technologies have applications in marketing, game design, and virtual reality.
c) Ethical Challenges
While generative AI offers immense potential, it also raises ethical concerns, including deepfakes, copyright issues, and misinformation. Addressing these challenges requires robust frameworks and regulation.
2. Neuromorphic Computing: Mimicking the Human Brain
Neuromorphic computing represents a revolutionary approach to AI by designing systems that mimic the human brain’s neural architecture. These systems aim to make AI more efficient, adaptable, and energy-conscious.
a) Architecture Inspired by Biology
Neuromorphic chips, such as Intel’s Loihi, use spiking neural networks (SNNs) to replicate the way neurons communicate in the brain. Unlike traditional digital systems, these chips process data in parallel, enhancing efficiency.
b) Real-World Applications
Neuromorphic AI is finding applications in robotics, sensory systems, and autonomous vehicles. For instance, these chips enable robots to learn tasks faster and adapt to changing environments with less computational power.
c) Energy Efficiency
A significant advantage of neuromorphic systems is their energy efficiency. By mimicking the brain’s low-power operation, these systems offer sustainable solutions for AI-driven applications, reducing environmental impact.
3. Explainable AI (XAI): Enhancing Transparency
As AI becomes increasingly complex, understanding its decision-making processes is critical. Explainable AI (XAI) focuses on making AI systems more transparent, interpretable, and trustworthy.
a) The Need for Explainability
In sensitive areas like healthcare and finance, stakeholders demand accountability for AI decisions. XAI addresses this by providing insights into how AI models reach conclusions, fostering trust and regulatory compliance.
b) Techniques in Explainability
- Feature Importance Analysis: Identifies which inputs are most significant to a model’s output.
- Local Interpretable Model-agnostic Explanations (LIME): Highlights factors influencing specific predictions.
- SHapley Additive exPlanations (SHAP): Quantifies the contribution of each input to a prediction.
c) Broad Implications
Explainable AI is critical for democratizing AI and ensuring equitable outcomes. By demystifying AI processes, XAI enables broader adoption and reduces biases in decision-making.
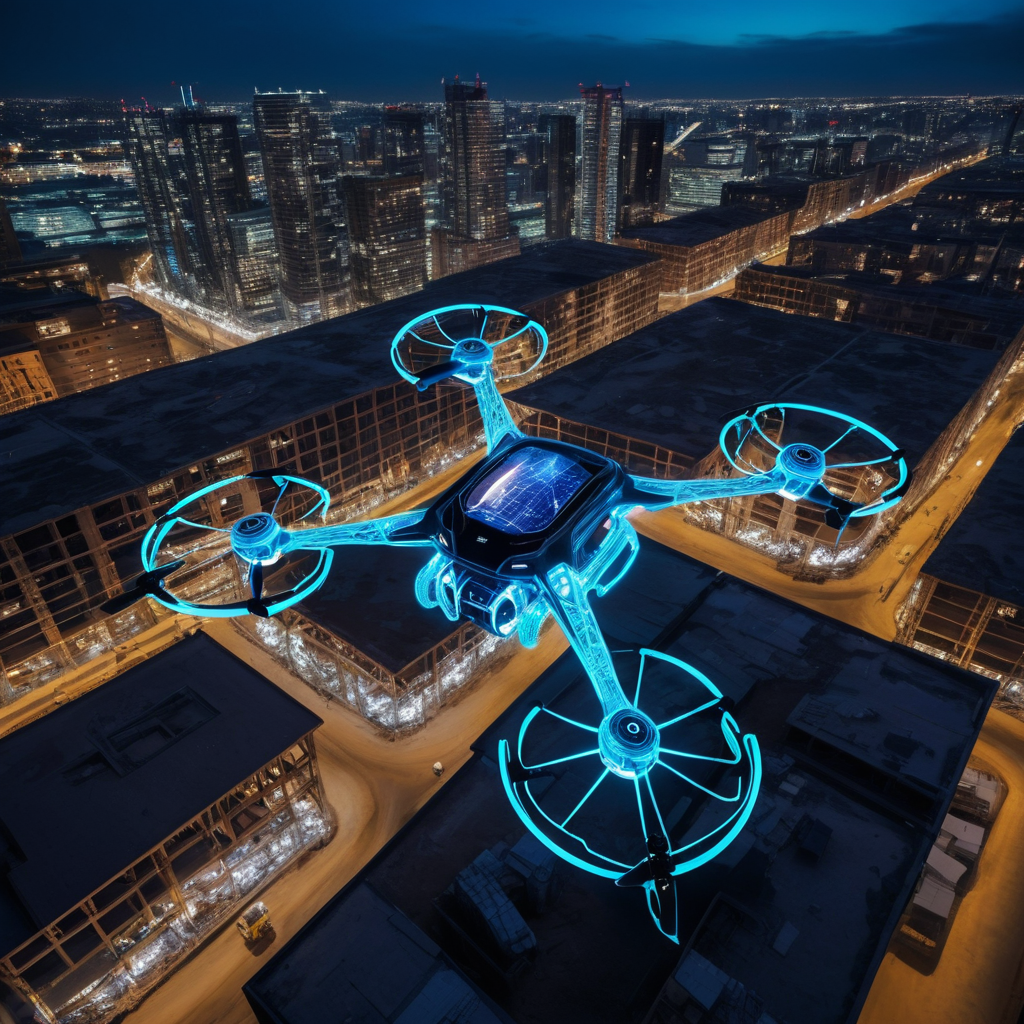
4. AI-Powered Edge Computing
Edge computing brings computational power closer to the source of data generation, enabling real-time processing and decision-making. Coupled with AI, edge computing offers enhanced performance, especially in latency-sensitive applications.
a) Decentralized AI Processing
AI at the edge reduces dependence on centralized cloud infrastructure. Devices like smart cameras, IoT sensors, and autonomous drones process data locally, ensuring faster responses and reduced bandwidth usage.
b) Applications Across Industries
- Healthcare: Wearable devices analyze patient data in real-time for personalized care.
- Retail: Smart shelves and inventory systems use edge AI to optimize stock management.
- Transportation: Autonomous vehicles rely on edge computing for instant navigation decisions.
c) Security and Privacy
Edge AI enhances data security by minimizing the need for data transfer to cloud servers. This approach ensures sensitive information remains on the device, aligning with privacy regulations like GDPR.
5. AI in Biotechnology: Revolutionizing Life Sciences
Emerging AI technologies are driving breakthroughs in biotechnology, transforming drug discovery, genetic research, and personalized medicine.
a) Accelerated Drug Discovery
AI models analyze vast datasets to identify potential drug candidates. Technologies like DeepMind’s AlphaFold have revolutionized protein structure prediction, accelerating the development of treatments for complex diseases.
b) Genomic Analysis
AI algorithms process genomic data to identify patterns and mutations associated with diseases. These insights enable researchers to develop gene-editing tools like CRISPR more effectively, advancing precision medicine.
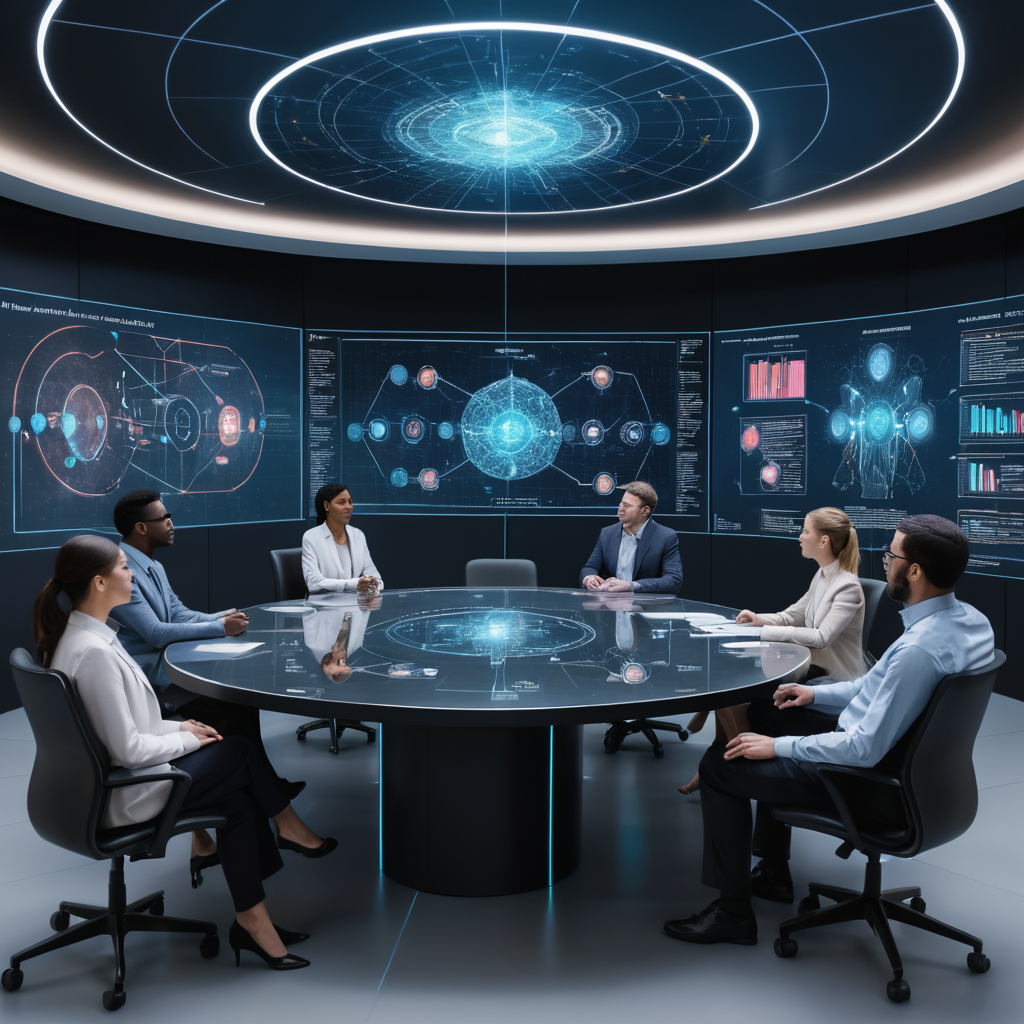
c) Synthetic Biology
AI assists in designing synthetic organisms and biomaterials for industrial, agricultural, and medical purposes. This emerging field holds promise for sustainable solutions to global challenges like food security and climate change.
6. Reinforcement Learning: Pioneering Autonomous Decision-Making
Reinforcement learning (RL) is a branch of AI where systems learn optimal actions by interacting with their environment. This technology is enabling advancements in robotics, gaming, and strategic planning.
a) Real-Time Adaptation
RL models learn from trial and error, adapting to dynamic scenarios. This capability makes RL ideal for training robots to perform complex tasks, such as assembly in manufacturing or exploration in hazardous environments.
b) Gaming and Simulation
Reinforcement learning has achieved milestones in gaming, with AI systems mastering games like chess, Go, and StarCraft. These achievements translate into real-world applications, such as training autonomous systems in simulated environments.
c) Collaborative AI
Emerging RL technologies focus on multi-agent systems, where AI agents work together to achieve shared goals. This approach is instrumental in developing swarm robotics and optimizing logistics networks.
Conclusion
Emerging AI technologies are charting new frontiers, offering transformative potential across industries and daily life. From generative AI and neuromorphic computing to explainable AI and biotechnology, these advancements promise to revolutionize how we live, work, and solve complex problems. However, their adoption must be accompanied by ethical considerations and robust governance to ensure they benefit humanity responsibly and sustainably.
C. Future Impacts of AI and ML
Artificial Intelligence (AI) and Machine Learning (ML) are poised to reshape the future in ways that are as profound as they are diverse. From revolutionizing industries to addressing global challenges, the advancements in AI and ML are set to drive transformative changes across every aspect of society. In this section, we explore the potential future impacts of AI and ML, focusing on their applications, benefits, and challenges.
1. Economic Transformation
AI and ML will redefine global economies by automating tasks, optimizing processes, and fostering innovation.
a) Automation and Job Evolution
AI-driven automation will handle repetitive tasks across industries, enabling human workers to focus on complex, creative, and strategic roles. For example:
- Manufacturing: Intelligent robots will streamline production lines.
- Healthcare: AI-powered diagnostics will assist doctors, enhancing efficiency.
- Customer Service: Chatbots will handle basic queries, leaving nuanced interactions for human agents.
b) Creation of New Job Markets
While automation might displace some roles, it will also create new opportunities in AI development, data analysis, and AI ethics oversight. The rise of AI-specific industries will demand specialized skill sets, spurring education and training programs.
c) Economic Growth
AI’s ability to optimize operations, predict market trends, and personalize consumer experiences will significantly boost productivity, driving global economic growth. A report by PwC predicts AI could contribute $15.7 trillion to the global economy by 2030.
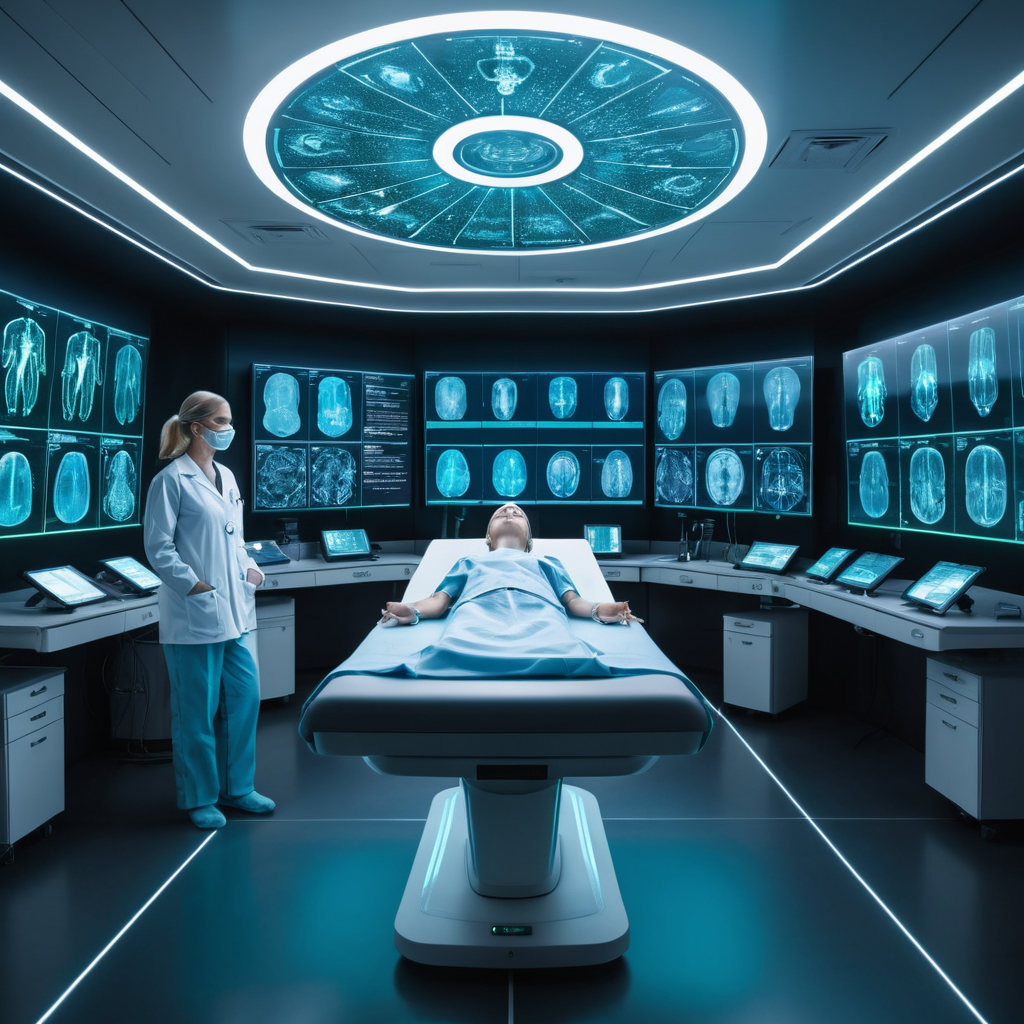
2. Advancements in Healthcare
The healthcare industry will be one of the biggest beneficiaries of AI and ML, with innovations improving patient outcomes, reducing costs, and enhancing access to medical services.
a) Personalized Medicine
AI algorithms will analyze genetic, lifestyle, and environmental data to create customized treatment plans for individual patients. This approach will improve treatment effectiveness while minimizing side effects.
b) Early Disease Detection
Machine learning models will identify patterns in medical imaging, lab results, and wearable device data, enabling early detection of diseases such as cancer, diabetes, and heart conditions.
c) Drug Discovery
AI will accelerate the development of new medications by predicting molecular behavior, simulating clinical trials, and optimizing drug formulations. Technologies like DeepMind’s AlphaFold are already revolutionizing protein structure prediction, a critical step in drug discovery.
d) Telemedicine and Remote Care
AI will enhance telemedicine platforms by analyzing patient data in real-time, recommending treatments, and enabling virtual health monitoring. This will increase access to healthcare for underserved populations.
3. Climate Change Mitigation
AI and ML will play a crucial role in combating climate change by optimizing resource use, predicting environmental changes, and driving sustainable practices.
a) Energy Efficiency
Smart grids powered by AI will optimize electricity distribution, reducing energy waste and integrating renewable energy sources like solar and wind into the power grid.
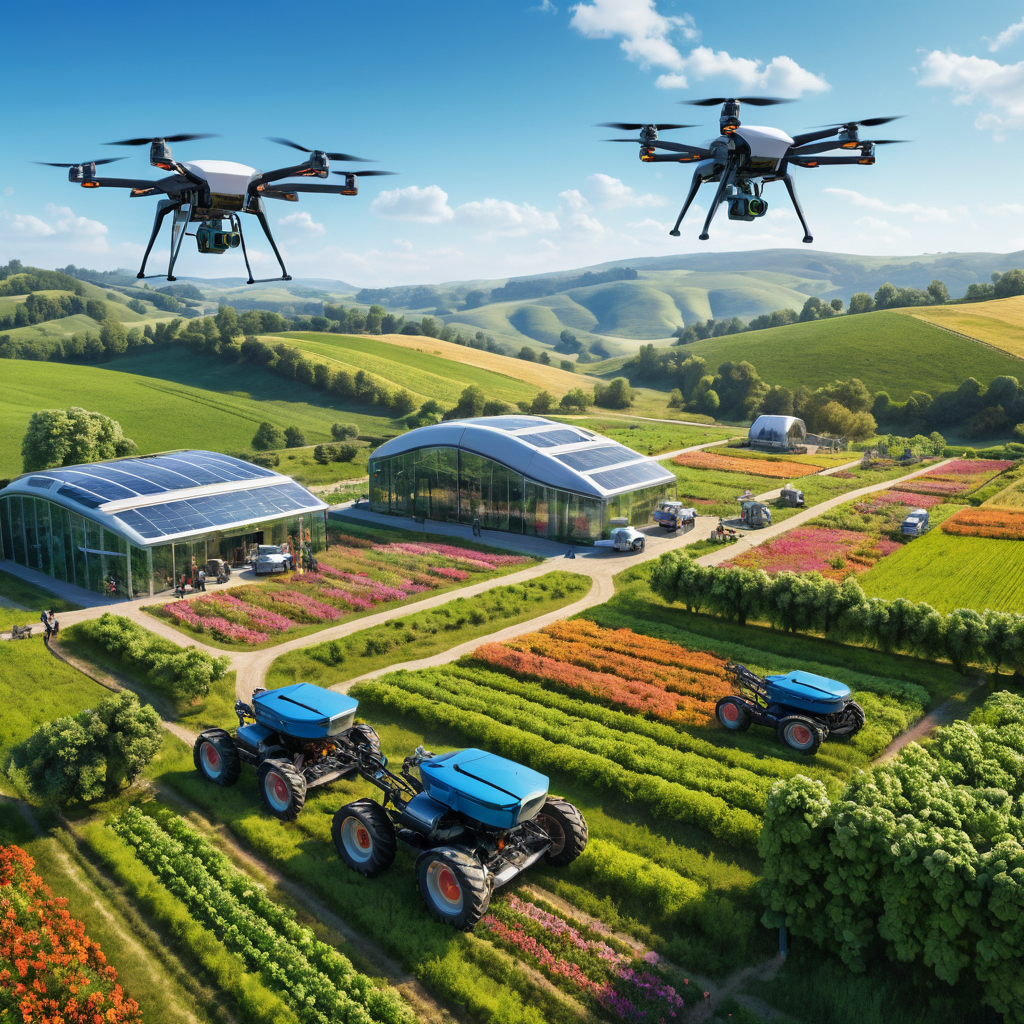
b) Environmental Monitoring
AI systems will analyze satellite imagery and sensor data to monitor deforestation, air and water quality, and wildlife populations. These insights will inform conservation efforts and policy decisions.
c) Sustainable Agriculture
AI-powered tools will optimize irrigation, monitor crop health, and reduce pesticide use, ensuring food security while minimizing environmental impact.
d) Disaster Prediction and Response
Machine learning models will predict natural disasters such as hurricanes, floods, and wildfires, enabling proactive measures to minimize damage and save lives.
4. Revolutionizing Education
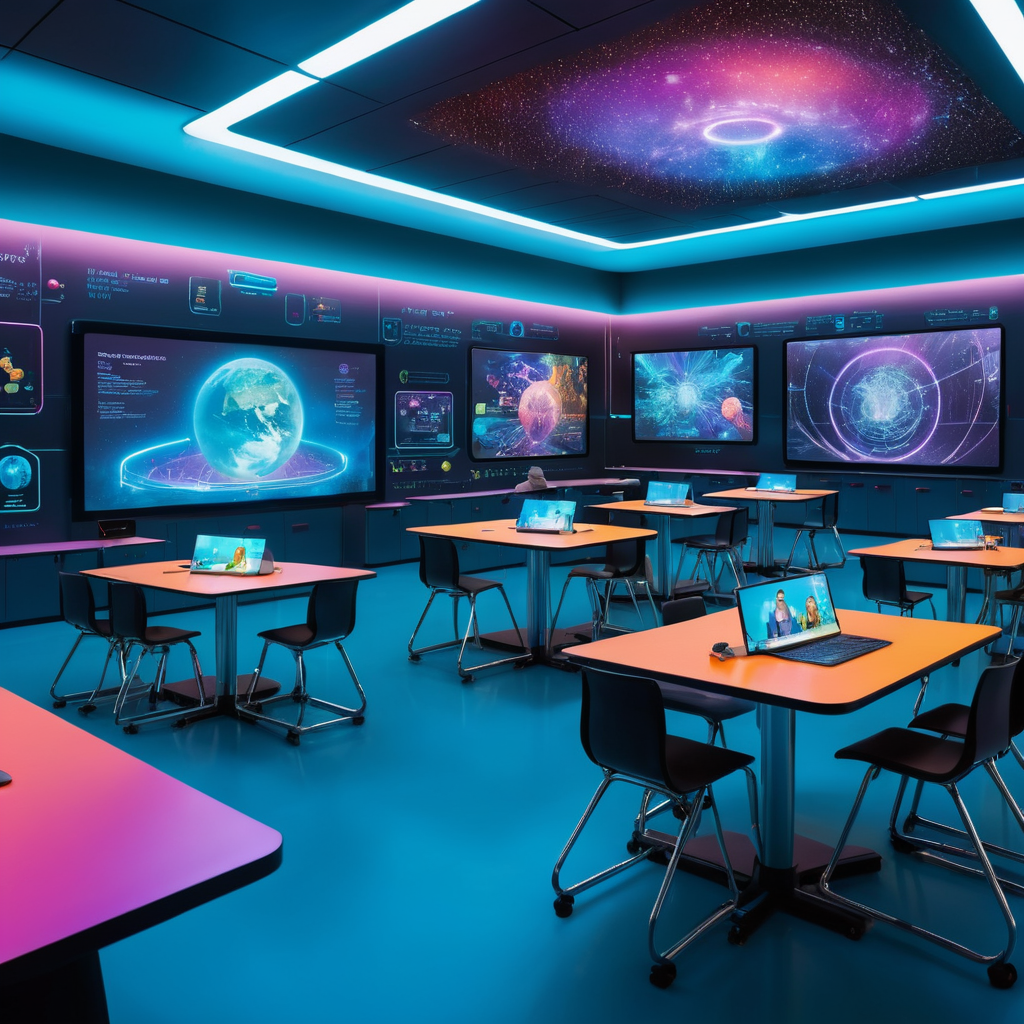
The integration of AI and ML in education will transform learning experiences, making them more personalized, accessible, and engaging.
a) Adaptive Learning Systems
AI-driven platforms will customize educational content based on individual learning styles, pacing, and interests, ensuring students achieve their full potential.
b) Virtual Tutors and Assistants
AI-powered virtual tutors will provide real-time feedback, answer questions, and support learners outside traditional classroom settings.
c) Inclusion and Accessibility
AI tools like speech recognition and translation software will make education accessible to people with disabilities and those in remote areas, bridging educational gaps globally.
d) Data-Driven Insights
AI will analyze student performance data to identify strengths, weaknesses, and trends, helping educators design more effective teaching strategies.
5. Ethical and Societal Considerations
As AI and ML become more pervasive, addressing ethical and societal implications will be critical to ensuring their benefits are maximized while minimizing potential harm.
a) Bias and Fairness
AI systems must be designed to avoid biases in decision-making. Diverse datasets, transparent algorithms, and rigorous testing will be essential to promoting fairness.
b) Privacy Concerns
With AI’s reliance on data, maintaining user privacy will be a priority. Implementing robust data encryption, consent mechanisms, and compliance with regulations like GDPR will be key.
c) Ethical Use Cases
AI developers and policymakers will need to establish ethical guidelines for AI applications, particularly in sensitive areas like surveillance, autonomous weapons, and social scoring.
d) Public Awareness and Trust
Building public trust in AI will require transparency, education, and open dialogue about its potential risks and benefits. AI literacy programs will empower people to navigate an AI-driven world responsibly.
6. Expanding Frontiers in Space Exploration
AI and ML will be instrumental in advancing space exploration by analyzing data, automating processes, and enabling deep space missions.
a) Autonomous Spacecraft
AI will allow spacecraft to make real-time decisions without human intervention, ensuring missions can adapt to unforeseen circumstances in deep space.
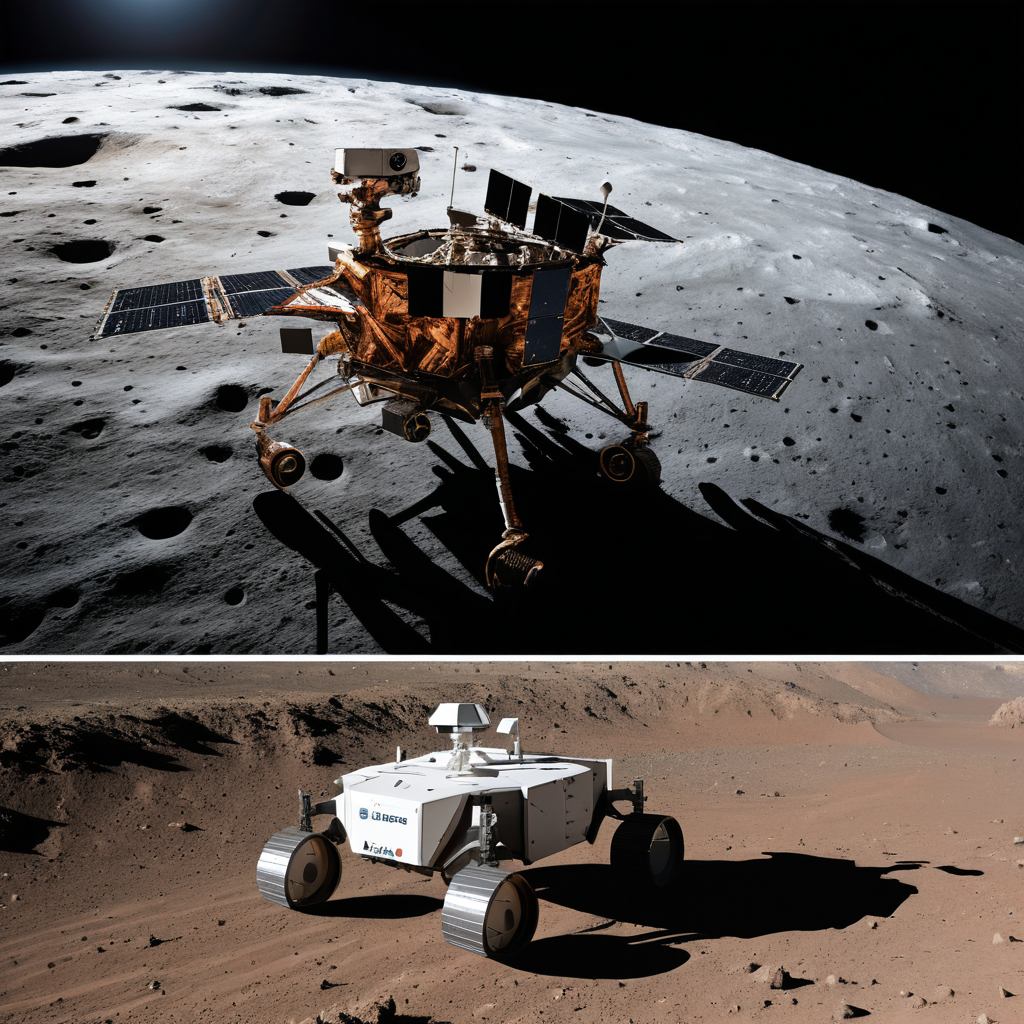
b) Planetary Exploration
Robots equipped with AI will explore planetary surfaces, analyzing soil, rocks, and atmospheric conditions to search for signs of life and resources.
c) Space Debris Management
AI systems will track and predict the movement of space debris, developing strategies to mitigate collisions and protect satellites.
d) Interstellar Communication
AI will enhance communication systems for interstellar exploration, optimizing data transmission and reducing signal delays.
Conclusion
The future impacts of AI and ML promise to be transformative, shaping the economy, healthcare, education, climate change efforts, ethical considerations, and space exploration. While these technologies hold immense potential, their integration must be accompanied by careful planning, robust ethical frameworks, and a commitment to inclusivity. By embracing these innovations responsibly, humanity can unlock a brighter, more sustainable future.
D. Challenges in AI Development
While Artificial Intelligence (AI) and Machine Learning (ML) hold transformative potential, their development is fraught with numerous challenges that must be addressed to unlock their full capabilities. These challenges span technical, ethical, societal, and regulatory dimensions, highlighting the complexities of integrating AI into everyday life and industries. Below, we delve into the critical challenges in AI development and their implications.
1. Technical Challenges
AI systems require significant computational resources, vast amounts of data, and robust algorithms. However, technical limitations often hinder development and deployment.
a) Data Dependency
AI and ML models depend heavily on large, high-quality datasets for training and accuracy. The scarcity of domain-specific or unbiased datasets can limit AI effectiveness. Additionally, data collection processes can be expensive and time-consuming, particularly in fields like healthcare or climate modeling.
b) Computational Complexity
Training AI models demands immense computational power, which can lead to high energy consumption and carbon footprints. For instance, training large language models like GPT-3 requires massive data centers and advanced hardware, creating barriers for smaller organizations with limited resources.
c) Generalization and Adaptability
Most AI systems excel in specific tasks but struggle to generalize across multiple domains. Building adaptable AI that can perform varied tasks without extensive retraining remains an elusive goal.
d) Interpretability and Explainability
Many AI models, especially deep learning systems, function as “black boxes,” making it difficult to understand their decision-making processes. This lack of transparency hinders trust, especially in critical areas like healthcare, finance, and law enforcement.
2. Ethical and Bias Concerns
AI’s growing presence raises ethical questions and concerns about bias, fairness, and societal impacts.
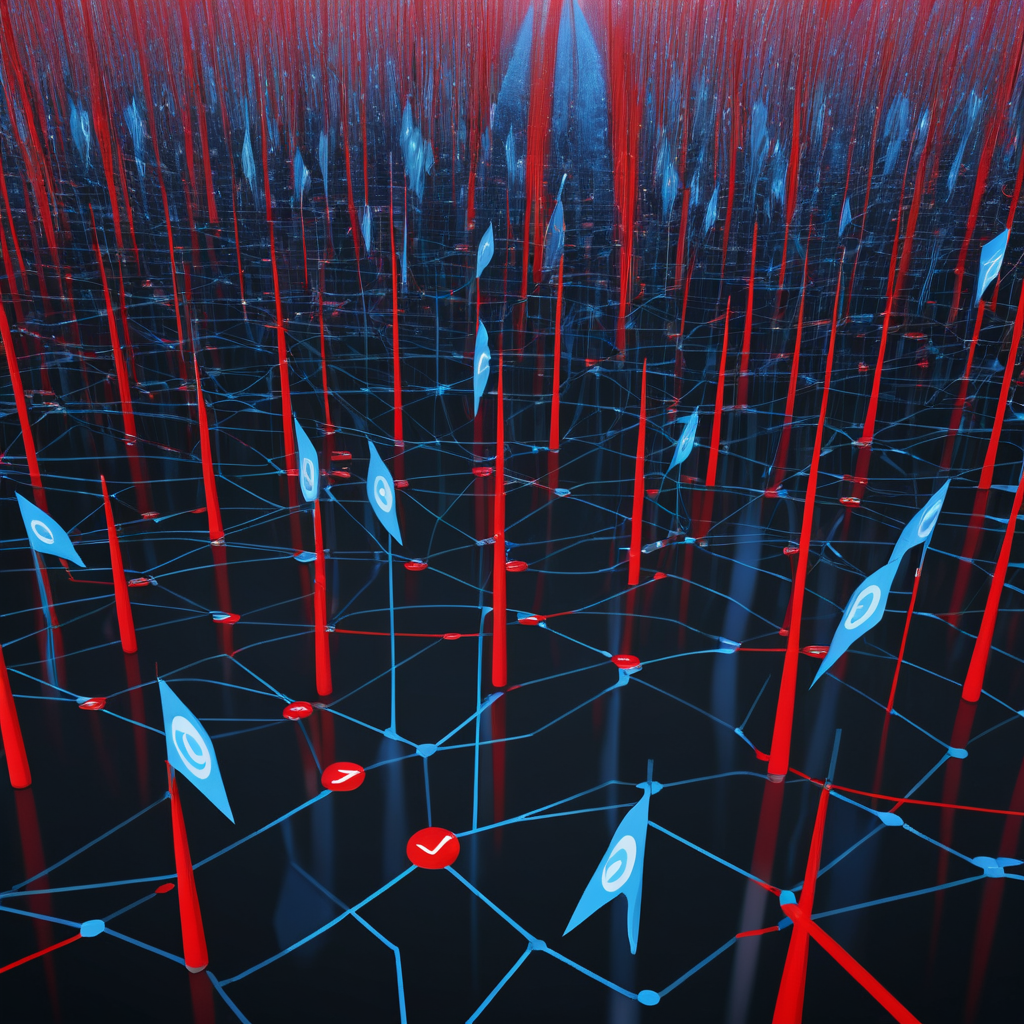
a) Algorithmic Bias
AI systems can inherit biases from the data they are trained on, leading to discriminatory outcomes in hiring, policing, or lending decisions. For instance, biased facial recognition systems have shown higher error rates for people of color, emphasizing the need for equitable data representation.
b) Privacy and Surveillance
AI technologies often require vast amounts of personal data, raising concerns about privacy and surveillance. Misuse of AI for mass surveillance, as seen in certain regions, threatens civil liberties and individual freedoms.
c) Ethical Dilemmas
Applications of AI in warfare, such as autonomous weapons, or social credit systems pose ethical dilemmas. Establishing clear boundaries for acceptable AI use is crucial to prevent misuse.
d) Accountability
Determining responsibility for AI-driven decisions remains challenging. When an AI system makes an error or causes harm, it is often unclear whether the developer, user, or system itself should be held accountable.
3. Societal Challenges
AI’s rapid adoption has far-reaching implications for society, including the workforce, education, and public trust.
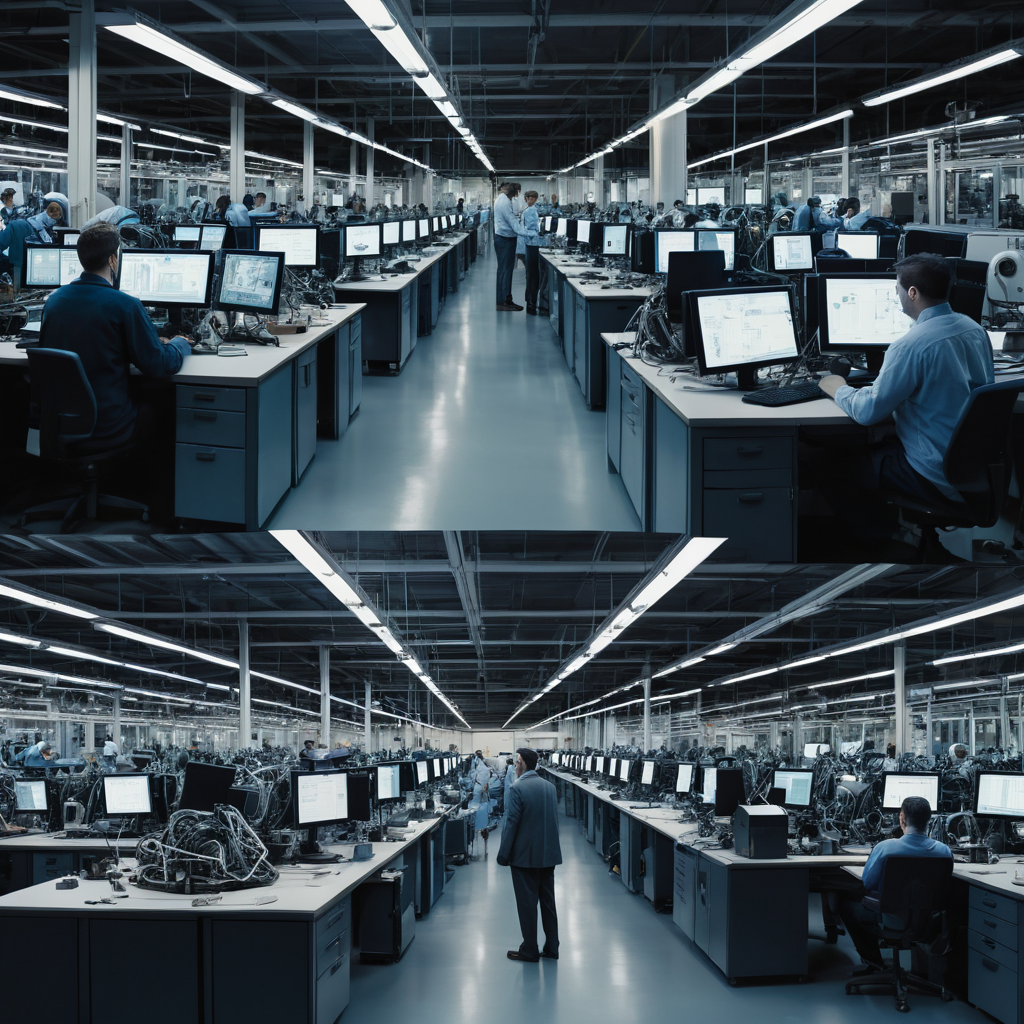
a) Job Displacement
While AI can create new job opportunities, it may also displace workers in roles that are easily automated. This shift requires reskilling initiatives to prepare workers for emerging AI-related jobs.
b) Public Misconceptions
AI is often misunderstood, with public fears fueled by dystopian portrayals in media. Educating people about the realities and limitations of AI is essential to fostering trust and responsible adoption.
c) Digital Divide
AI development and adoption can exacerbate inequalities between regions with advanced technologies and those without access to AI resources. Bridging this digital divide is critical to ensuring inclusive AI benefits.
d) Ethical Implications of Deepfakes
The misuse of AI to create deepfakes—highly realistic but false audio or video—poses risks to privacy, misinformation, and public trust. Combating this misuse requires advanced detection tools and regulatory frameworks.
4. Regulatory and Policy Challenges
The rapid evolution of AI technology often outpaces the development of policies and regulations needed to govern its use responsibly.
a) Lack of Standardized Frameworks
Countries and organizations vary in their approach to AI regulation. This inconsistency creates challenges for international collaboration and hinders the establishment of universal AI standards.
b) Intellectual Property Issues
Determining intellectual property rights for AI-generated content or inventions is a growing concern. Clear legal guidelines are needed to address ownership and copyright issues.
c) Cross-Border Data Flow
AI systems often rely on data that crosses international boundaries, raising concerns about data sovereignty and compliance with diverse data protection laws like GDPR or CCPA.
d) Ethical AI Development Guidelines
While several organizations advocate for ethical AI principles, implementing these guidelines effectively in diverse cultural and industrial contexts remains a challenge.
5. Security Threats
AI technologies, while powerful, are also vulnerable to misuse and cyberattacks.
a) Adversarial Attacks
AI models can be manipulated with adversarial inputs—carefully crafted data that causes the model to make incorrect decisions. For example, adversarial attacks on self-driving cars could lead to accidents.
b) AI-Powered Cyberattacks
Malicious actors can use AI to launch sophisticated cyberattacks, such as phishing campaigns that mimic human behavior or automated hacking tools that exploit vulnerabilities.
c) Robustness and Resilience
Ensuring AI systems remain robust against disruptions or malicious attempts is crucial, especially in critical sectors like defense, healthcare, and finance.
6. Environmental Concerns
The energy consumption associated with AI development is a growing concern, particularly given its implications for climate change.
a) Carbon Footprint
Training large AI models requires significant computational power, leading to high energy usage. For instance, the carbon footprint of training GPT-3 is comparable to the annual emissions of several cars.
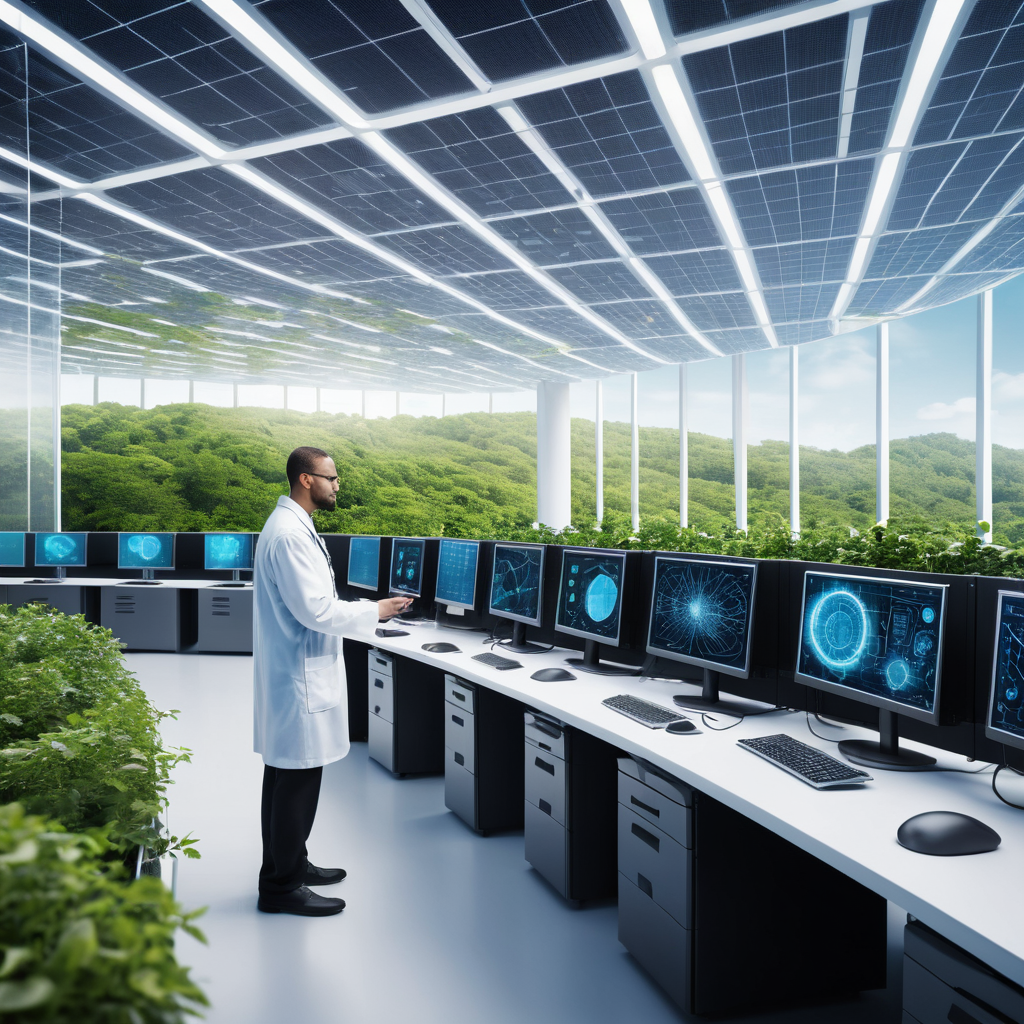
b) Sustainable AI Development
Developing energy-efficient algorithms and hardware, as well as utilizing renewable energy for data centers, will be essential to minimizing AI’s environmental impact.
Conclusion
Addressing these challenges is crucial to realizing the full potential of AI while mitigating its risks. Collaboration among governments, researchers, industry leaders, and the public will be essential to overcoming technical, ethical, societal, and regulatory hurdles. By fostering innovation responsibly and inclusively, humanity can ensure that AI contributes positively to global progress.
2. Quantum Computing: Unlocking Unprecedented Computational Power
Quantum computing represents one of the most revolutionary advancements in the field of technology, promising to solve problems far beyond the capacity of classical computers. Built on the principles of quantum mechanics—such as superposition, entanglement, and quantum tunneling—quantum computers can perform calculations at speeds unimaginable with traditional systems. While classical computers rely on bits to process data in binary (0s and 1s), quantum computers use quantum bits, or qubits, which can exist in multiple states simultaneously. This property enables quantum computers to process vast amounts of data in parallel, unlocking new possibilities across industries.
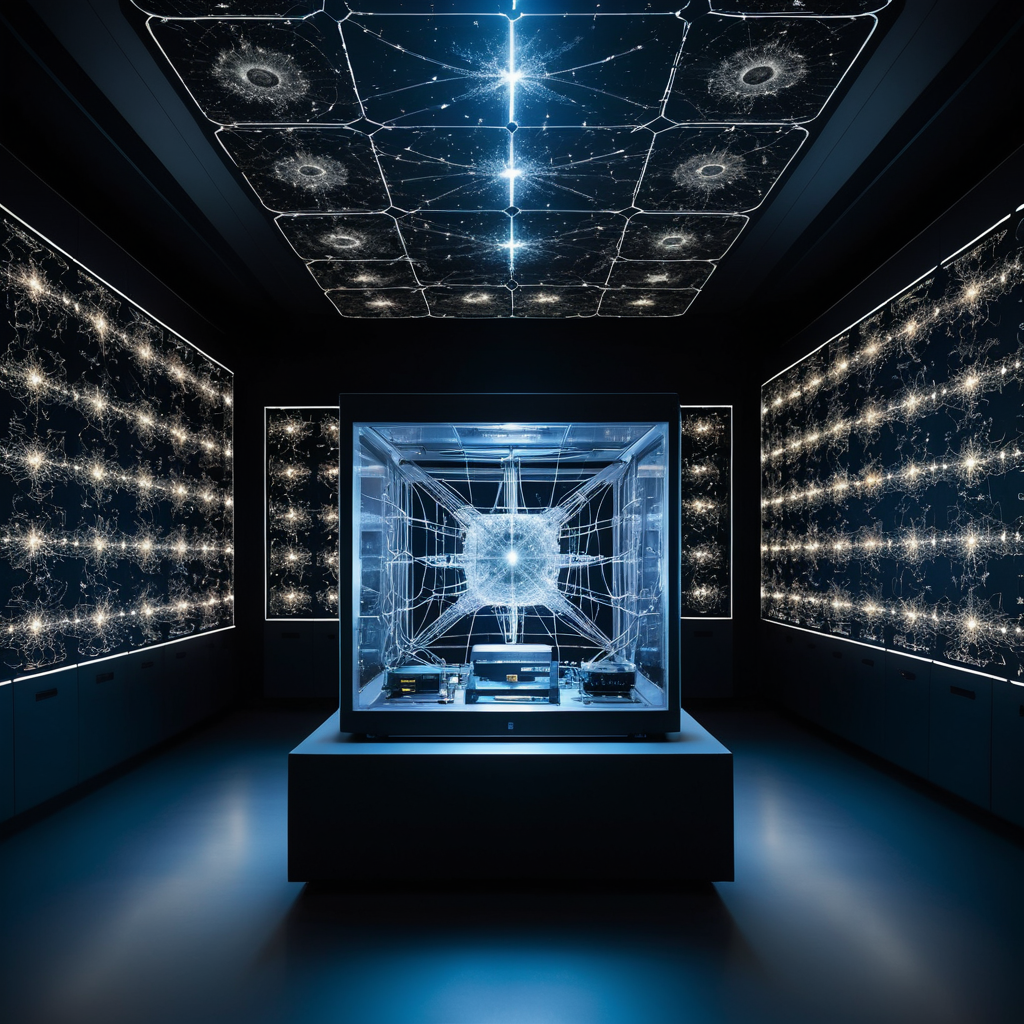
A. Current State of Quantum Computing
Quantum computing, although still in its nascent stages, has made remarkable strides over the past few years. The realm of quantum computing represents a frontier of innovation that promises to revolutionize industries, from cryptography to drug discovery. Today, quantum computing is primarily in the research and experimental phase, with multiple challenges to overcome before its full potential can be realized. However, advancements in quantum hardware, algorithms, and practical applications have already begun to show what could be possible in the near future.
1. Technological Progress
In the past decade, the progress in quantum computing has been staggering, with several companies and research institutions unveiling impressive breakthroughs. However, the quantum computing ecosystem remains fragmented, with multiple approaches being pursued by different organizations. The primary challenge facing quantum computing technology is the development of scalable, stable, and reliable quantum processors capable of outperforming classical computers in real-world tasks.
a) Quantum Hardware Developments
Various approaches to quantum hardware exist, with the two most prominent being superconducting qubits and trapped ions. Superconducting qubits are the approach adopted by companies like IBM, Google, and Rigetti Computing. These qubits are based on superconducting circuits, which can carry current without resistance at extremely low temperatures. Google’s Sycamore processor, for example, achieved quantum supremacy in 2019 by solving a problem in 200 seconds that would take classical supercomputers thousands of years to complete.
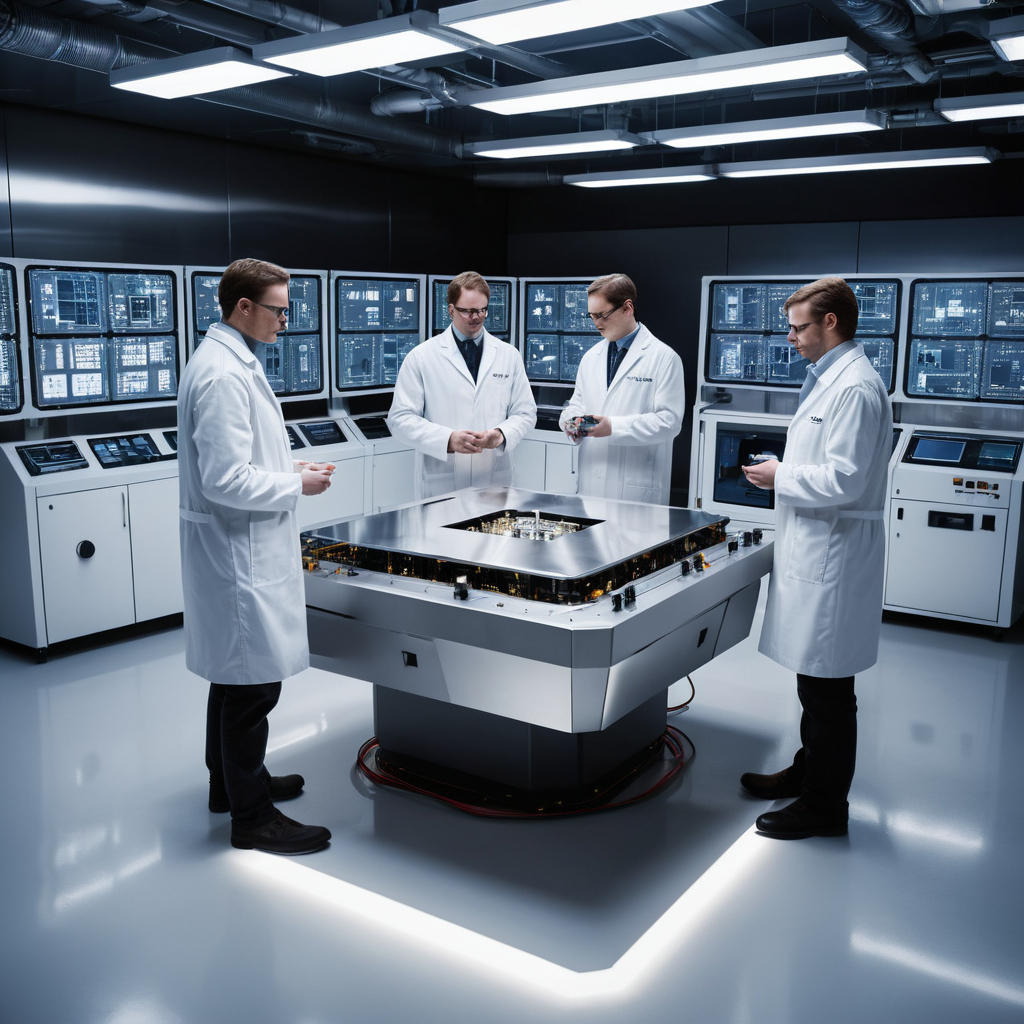
Trapped-ion qubits are another significant quantum hardware approach, with companies like IonQ and Honeywell at the forefront. Trapped ions are charged atoms confined in electromagnetic fields. These ions are manipulated by lasers, allowing them to function as qubits. The advantage of this method is its scalability, precision, and ability to maintain quantum coherence for longer durations. IonQ’s quantum systems are already accessible via the cloud, enabling broader access to quantum computing.
b) Quantum Computing as a Service
To make quantum computing more accessible, cloud-based quantum computing platforms have emerged, offering businesses and researchers the opportunity to run quantum algorithms on remote quantum processors. IBM’s Quantum Experience, for instance, allows users to run quantum algorithms on IBM’s quantum hardware via the cloud. Similarly, Amazon Braket provides a platform for developers to experiment with quantum algorithms using hardware from various quantum computing companies.
This democratization of quantum computing allows researchers without access to quantum hardware to explore its potential, thus accelerating the development of quantum applications across different industries. The ability to access quantum computing remotely has opened new avenues for collaboration and innovation in the quantum space.
2. Applications in Research
Though quantum computing is still in its infancy in terms of mainstream applications, several industries and research fields are already beginning to explore its potential. The promise of quantum computing lies in its ability to solve complex problems that are currently beyond the reach of classical computing. Some of the primary areas of research where quantum computing is making an impact include cryptography, materials science, drug discovery, and optimization.
a) Cryptography
Quantum computing’s potential to break traditional encryption schemes, such as RSA encryption, has garnered significant attention. Shor’s algorithm, a quantum algorithm developed in the 1990s, can efficiently factor large numbers, threatening the security of modern cryptographic systems. However, quantum computing also offers the opportunity to create quantum-resistant encryption protocols. One of the most promising areas of quantum encryption is Quantum Key Distribution (QKD), which uses the principles of quantum mechanics to securely share encryption keys over long distances. ID Quantique, a leader in quantum cryptography, is already providing QKD solutions for secure communications in government and financial sectors.
b) Drug Discovery and Healthcare
In the field of healthcare, quantum computing holds immense potential in revolutionizing drug discovery and personalized medicine. Quantum computers can simulate the behavior of molecules at the quantum level, which is beyond the capability of classical supercomputers. This allows researchers to design and test new drugs in a fraction of the time it would take with conventional methods.
Companies such as D-Wave and Microsoft’s Quantum Lab are exploring quantum algorithms that can optimize molecular simulations for drug discovery. For example, the discovery of new pharmaceuticals for conditions like cancer or Alzheimer’s disease could be expedited by leveraging quantum simulations to predict how molecules will interact. Quantum computing could also play a vital role in personalized medicine by simulating an individual’s unique biological makeup and predicting the most effective treatments.
c) Optimization Problems
Optimization is another area where quantum computing shows great promise. Quantum computers excel at solving optimization problems that involve evaluating numerous variables simultaneously, such as supply chain management, traffic flow optimization, and financial portfolio optimization. Quantum algorithms like Grover’s algorithm can significantly speed up searching through large datasets, which has important implications for industries ranging from logistics to finance.
Volkswagen, for example, is exploring quantum computing to optimize traffic flow in urban areas, reducing congestion and improving efficiency. Similarly, in finance, quantum computing could improve the modeling and prediction of financial markets, leading to better risk management strategies and more efficient portfolio allocations.
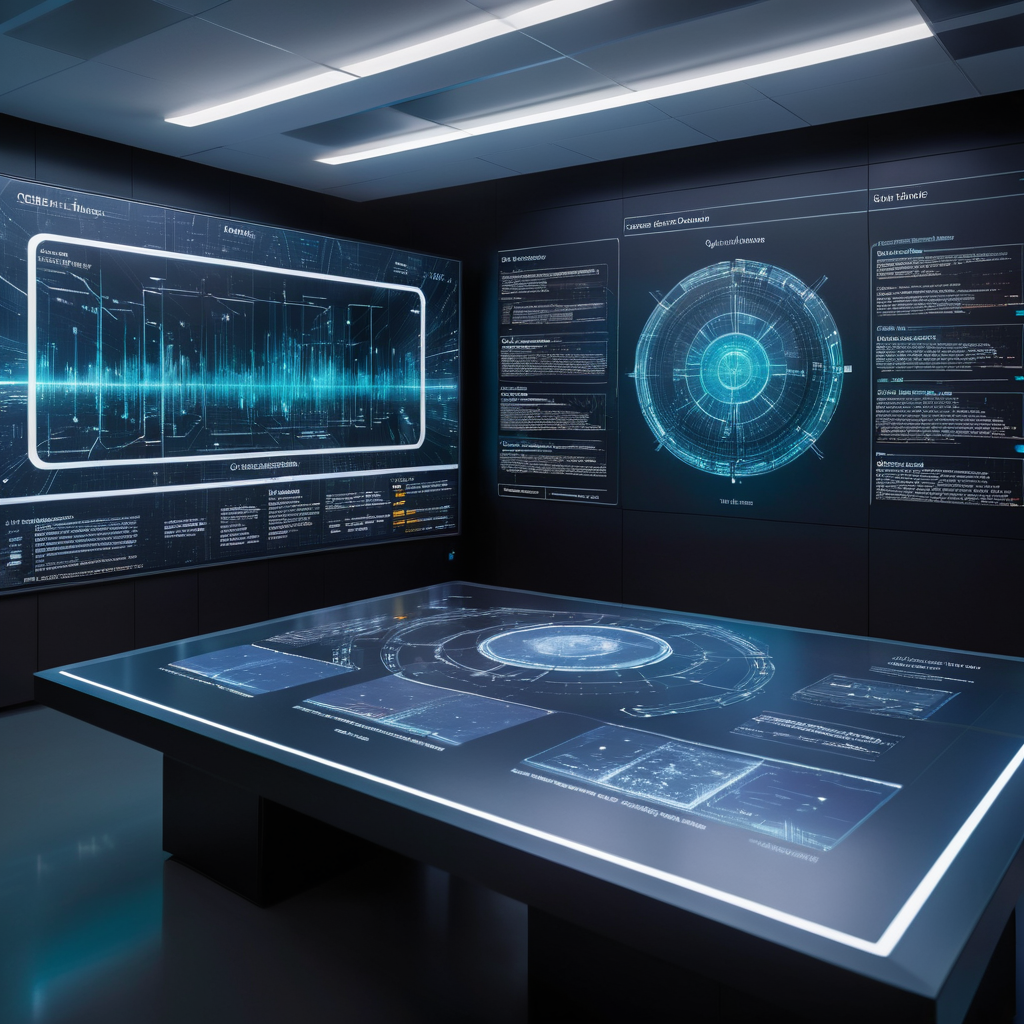
3. Quantum Algorithms
A major driver of quantum computing’s potential is the development of quantum algorithms, which are designed to harness the unique properties of quantum mechanics to perform computations much faster than classical algorithms. These algorithms are central to quantum computing’s promise to solve problems that were once considered intractable.
a) Shor’s Algorithm
One of the most well-known quantum algorithms is Shor’s algorithm, which allows for the efficient factorization of large numbers. This is significant because the security of many encryption systems, including RSA encryption, relies on the difficulty of factoring large prime numbers. Shor’s algorithm demonstrates that quantum computers can potentially break current cryptographic systems, necessitating the development of quantum-resistant encryption methods.
b) Grover’s Algorithm
Another notable quantum algorithm is Grover’s algorithm, which provides a quadratic speedup for unstructured search problems. In classical computing, searching through an unsorted database requires examining each entry one by one. However, Grover’s algorithm allows quantum computers to search through an unsorted database in roughly the square root of the number of entries, dramatically reducing the time required for search operations.
c) Quantum Machine Learning (QML)
Quantum computing’s integration with machine learning has given rise to the field of Quantum Machine Learning (QML). QML leverages quantum algorithms to enhance machine learning processes, enabling faster training and more accurate models. For instance, quantum computers could improve the performance of artificial neural networks, natural language processing tasks, and predictive analytics. Research in QML is still in its early stages, but it holds tremendous potential for fields such as AI, image recognition, and medical diagnosis.
Conclusion
The current state of quantum computing is marked by significant breakthroughs in hardware development, the rise of cloud-based quantum services, and increasing exploration of its applications in various sectors. While the technology is still evolving and facing challenges in scalability, error correction, and practical implementation, its potential is undeniable. As we look toward the next decade, continued advancements in quantum hardware, algorithms, and applications will be crucial in unlocking its transformative power. The ongoing research and collaboration between industry giants and academic institutions will drive quantum computing to new heights, reshaping industries and solving some of the world’s most complex problems.
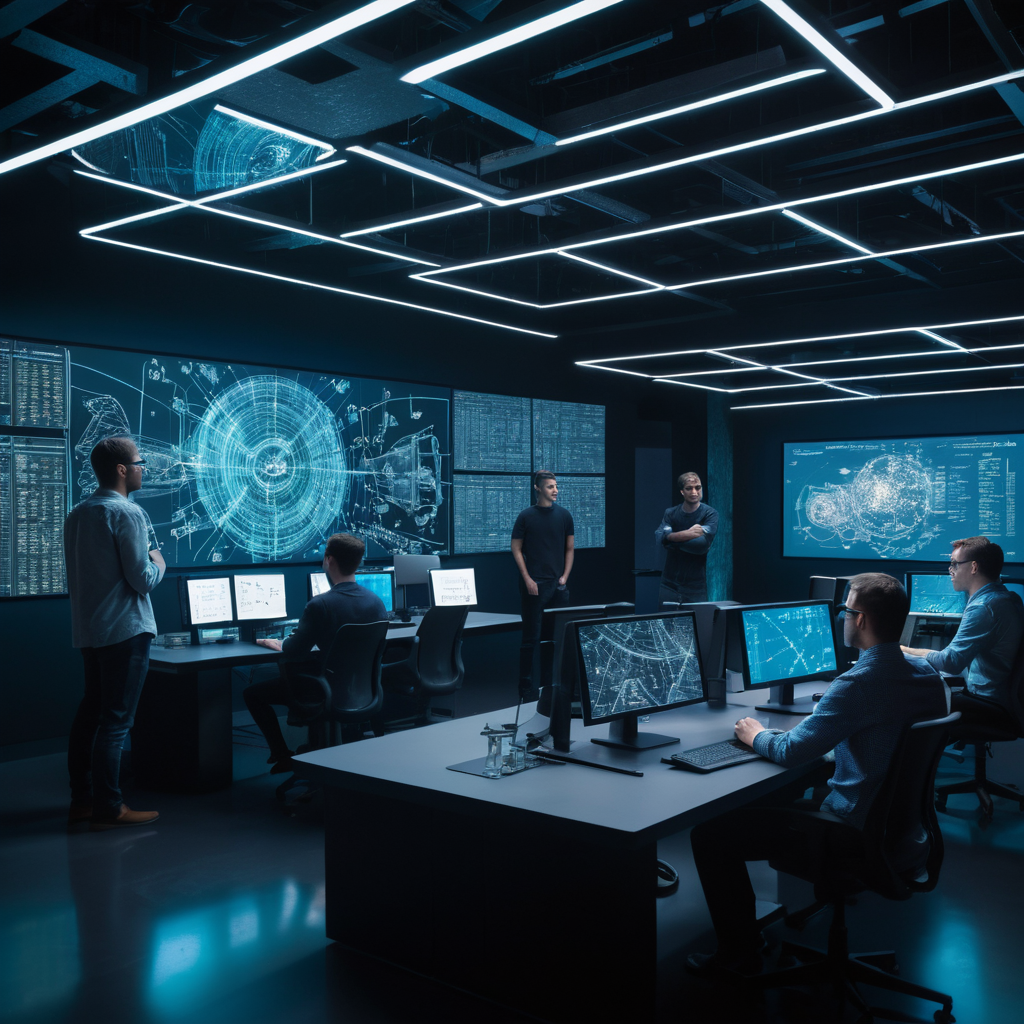
B. Future Potential of Quantum Computing
Quantum computing has garnered significant attention due to its transformative potential across numerous industries. Although we are still in the early stages, the future of quantum computing promises to reshape sectors such as finance, healthcare, cryptography, and artificial intelligence. The capability to solve problems that were previously thought to be computationally intractable is just the beginning. As quantum technologies evolve, they hold the promise of solving some of the world’s most pressing challenges, from accelerating drug development to advancing climate change solutions. However, realizing this potential will require overcoming significant technical hurdles, and it is essential to understand what the future might look like.
1. Quantum Supremacy and Practical Use Cases
One of the most significant milestones anticipated in quantum computing is the achievement of quantum supremacy—a point at which quantum computers can outperform classical supercomputers on certain tasks. Google’s 2019 achievement with the Sycamore processor marked a crucial step toward this goal, as it demonstrated that quantum computers could solve problems in seconds that would take traditional supercomputers thousands of years to solve.
While quantum supremacy in some applications is now a reality, the real potential lies in scaling quantum computing for practical, real-world applications. A major challenge for the future is to find tasks that quantum computers can solve that are both useful and surpass classical computers’ capabilities. Fields like cryptography, material science, drug development, and optimization problems represent the primary areas where quantum computing can have a tangible, impactful role.
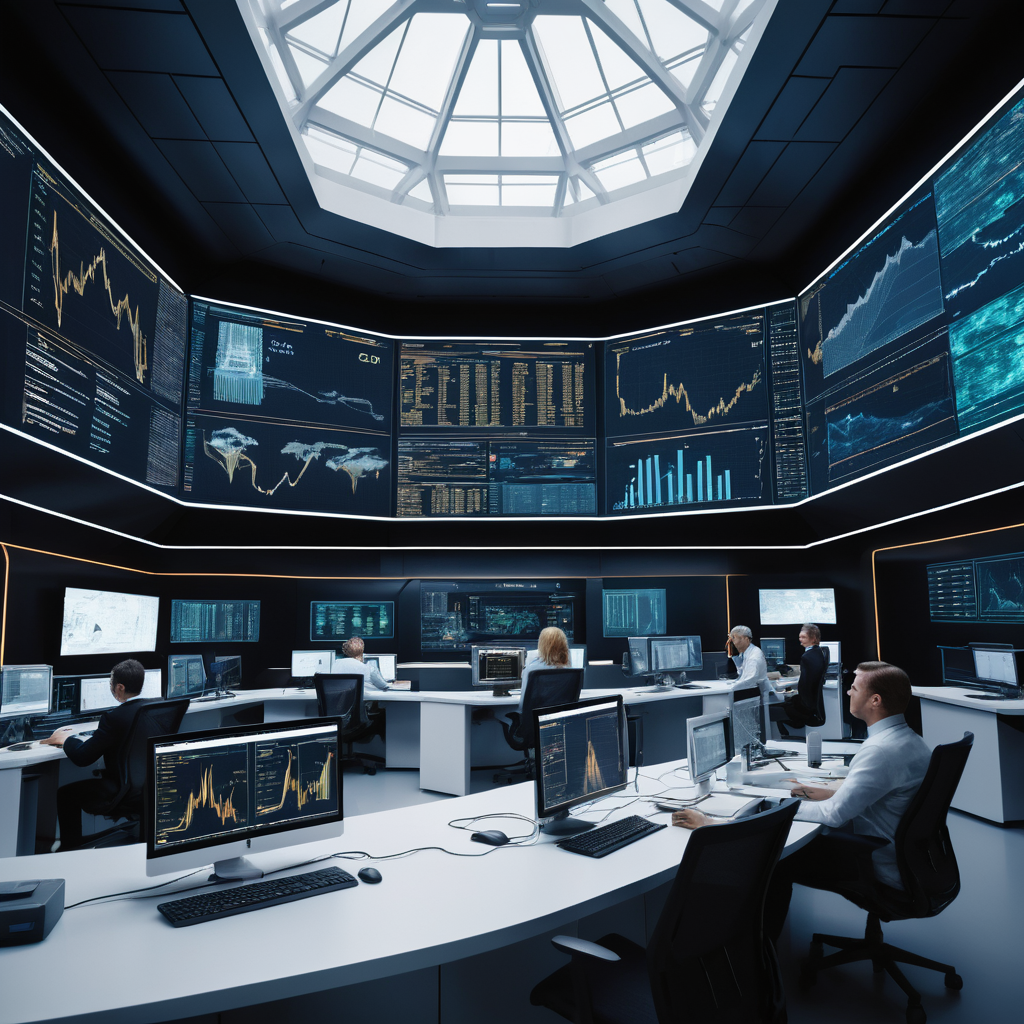
a) Cryptography and Data Security
Quantum computing’s potential to revolutionize cryptography is profound. While current encryption methods rely on the difficulty of factoring large numbers, quantum computers can leverage algorithms like Shor’s algorithm to break traditional encryption systems. This presents a major challenge for data security across the world, but it also opens the door for more advanced forms of encryption, such as quantum key distribution (QKD).
QKD uses quantum mechanics to create unbreakable encryption channels, which will be essential for protecting sensitive information in a world where quantum computers are commonplace. As quantum computing advances, industries like finance, defense, and healthcare will need to adopt new quantum-resistant algorithms to safeguard their data.
b) Drug Discovery and Healthcare
In the future, quantum computing will revolutionize healthcare by making it possible to perform quantum simulations of molecules and chemical reactions at a level of detail never before achievable. Classical computers often struggle to simulate large molecules or understand complex biological systems, which makes drug discovery an expensive and time-consuming process.
Quantum computing’s ability to simulate molecular structures in real-time could drastically shorten the time required to develop drugs, particularly in areas like cancer, Alzheimer’s disease, and antiviral medications. By simulating the interaction between different molecules, quantum computers can identify promising drug candidates, reducing the need for lengthy laboratory trials.
For personalized medicine, quantum computing will help tailor medical treatments to individual genetic profiles, optimizing therapies and improving outcomes. With the ability to run simulations for millions of patients simultaneously, quantum computing could drive the next era of personalized healthcare, leading to treatments that are more effective and targeted.
2. Quantum Machine Learning (QML) and Artificial Intelligence
The integration of quantum computing with machine learning (ML) has given rise to Quantum Machine Learning (QML). Quantum computers can process vast amounts of data exponentially faster than classical computers, making them ideal for improving the efficiency and accuracy of machine learning models.
a) Accelerating Artificial Intelligence
Quantum computing’s ability to analyze large datasets and recognize patterns at unprecedented speeds can significantly enhance artificial intelligence systems. Classical machine learning algorithms, while powerful, are limited by the computational power available on traditional hardware. However, quantum machine learning algorithms can potentially revolutionize fields such as natural language processing (NLP), computer vision, and reinforcement learning.
Quantum computers can also enhance deep learning models, which involve multiple layers of neural networks. These models typically require massive datasets and substantial computational power, which quantum machines are well-equipped to handle. For example, quantum annealing, a method used to solve optimization problems, could optimize the training process of neural networks, leading to better and faster results.
b) Quantum-enhanced Data Analysis
Data analysis, which lies at the heart of machine learning, is expected to be greatly enhanced by quantum computing. The ability to process large datasets at a quantum level means that businesses and organizations can make more accurate predictions and insights based on complex data. This has profound implications for sectors like finance, marketing, and cybersecurity.
For example, quantum computers could significantly improve anomaly detection in cybersecurity by analyzing network traffic patterns and identifying malicious activity more quickly than current methods allow. Similarly, quantum-enhanced machine learning could optimize investment portfolios, predict consumer behavior with higher accuracy, and detect fraud with unprecedented precision.
3. Advances in Materials Science and Sustainability
Quantum computing will also play a pivotal role in the discovery of new materials and solutions for sustainability challenges. One of quantum computing’s most exciting potential applications lies in solving complex problems related to materials science.
a) Designing New Materials
At the molecular and atomic level, quantum computers can simulate the behavior of materials with extreme accuracy. For instance, quantum computers could predict the properties of materials that have not yet been discovered. These new materials could have a wide range of applications, from superconductors to advanced batteries, offering improvements in efficiency and performance in many industries.
The potential to develop new superconducting materials—which allow electricity to flow without resistance at higher temperatures—could lead to innovations in energy transmission, reducing energy losses in power grids. Similarly, quantum computing could accelerate the discovery of more efficient solar cells, leading to cheaper and more sustainable energy solutions.
b) Addressing Climate Change
In the fight against climate change, quantum computing could provide significant contributions. For example, quantum simulations could help researchers identify better catalysts for carbon capture, leading to more efficient methods for reducing greenhouse gases in the atmosphere. Furthermore, quantum models could aid in the development of more effective materials for solar panels, energy storage systems, and hydrogen fuel cells, helping to reduce the world’s dependence on fossil fuels.
Quantum simulations can also improve weather prediction models, allowing for more accurate climate modeling. This could enhance disaster preparedness, as better predictive models will allow governments and organizations to respond more effectively to natural disasters like hurricanes, floods, and wildfires.
4. Quantum Computing for Optimization
Optimization problems are pervasive in every industry, from logistics and supply chain management to financial modeling and manufacturing. Quantum computing offers a dramatic improvement in solving complex optimization problems, which involve finding the best solution out of a large number of possibilities. These problems often require vast amounts of computational power and time, making them well-suited for quantum computers.
a) Supply Chain Optimization
Quantum computing has the potential to revolutionize supply chain management by providing real-time, optimized routes for transporting goods. Traditional optimization methods, which rely on classical computers, can only evaluate a limited number of possibilities at a time. However, quantum computers can evaluate many variables simultaneously, allowing for more efficient and cost-effective supply chain decisions.
In addition, quantum computing can optimize inventory management and manufacturing schedules, ensuring that resources are allocated most efficiently. This will be especially important in industries like e-commerce and logistics, where supply chain efficiency is critical to maintaining a competitive edge.
b) Financial Portfolio Optimization
In finance, quantum computing’s ability to solve complex optimization problems could drastically improve portfolio management. Classical computers can only evaluate a limited number of investment strategies, but quantum computers could assess thousands of potential portfolios in a fraction of the time. This could lead to more effective strategies for minimizing risk while maximizing returns, ultimately transforming investment management and financial analysis.
Conclusion: The Path to Quantum Advantage
The future potential of quantum computing is immense, but realizing this potential will require overcoming technical challenges in hardware, error correction, and algorithm development. Despite these challenges, quantum computing will likely play a transformative role in fields like cryptography, drug discovery, machine learning, sustainability, and optimization. As quantum hardware and algorithms continue to evolve, we can expect breakthroughs that will not only push the boundaries of what’s computationally possible but also address some of humanity’s most pressing challenges, from combating climate change to revolutionizing healthcare.
C. Key Players in Quantum Computing
As quantum computing continues to evolve from theory to reality, numerous organizations, from tech giants to academic institutions and startups, are making significant strides in research and development. The quantum computing ecosystem includes a wide range of stakeholders—each contributing to the technology’s advancement in unique ways. These players are critical in driving quantum research, developing hardware, creating software solutions, and exploring the vast potential applications of quantum computing.
The key players in quantum computing can be broadly categorized into corporations, research institutions, and startups. Each of these groups brings different expertise and resources, contributing to various facets of quantum computing’s progress.
1. Technology Giants Leading Quantum Development
Tech giants such as IBM, Google, Microsoft, and Intel have made significant investments in quantum computing research and development. These companies are developing both quantum hardware and software platforms, with an eye toward practical, large-scale implementations of quantum computers.
a) IBM’s Quantum Journey
IBM has been at the forefront of quantum computing research for many years. The company’s IBM Quantum initiative is a key example of its commitment to leading the industry. IBM has developed a quantum computing platform called Qiskit, which provides an open-source framework for quantum programming and simulation. IBM’s quantum research is focused on building scalable quantum computers that can solve real-world problems in fields like optimization, machine learning, and cryptography.
In addition to hardware development, IBM’s quantum cloud service allows users to access quantum computers via the cloud. This service, known as IBM Quantum Experience, enables researchers and developers to test and experiment with quantum algorithms, providing access to quantum hardware without the need for extensive physical infrastructure. The company is also working on error-correction techniques and developing new materials for quantum processors.
b) Google’s Quantum Leap
Google made headlines in 2019 with its landmark achievement of quantum supremacy, wherein its Sycamore quantum processor outperformed traditional supercomputers in a specific computational task. This marked a major breakthrough in quantum computing. Google’s goal is to build universal quantum computers that can solve a wide variety of problems, including cryptography, material science, and machine learning.
Google’s Quantum AI division is focused on scaling up quantum systems and improving quantum circuits. The company is also exploring the intersection of quantum machine learning and artificial intelligence, where quantum computers could revolutionize how AI models are trained and optimized.
c) Microsoft and Azure Quantum
Microsoft’s approach to quantum computing is centered around its Azure Quantum platform. Rather than focusing solely on hardware, Microsoft aims to provide a cloud-based quantum ecosystem that integrates with existing classical computing systems. The company is developing a unique quantum computing architecture based on topological qubits, which are more stable than traditional qubits, making them less susceptible to errors.
Additionally, Microsoft has created a quantum programming language called Q#, which allows developers to create quantum algorithms. The Azure Quantum platform provides access to multiple quantum hardware providers, including Honeywell and IonQ, allowing users to run their quantum programs on the best available quantum systems.
d) Intel’s Quantum Computing Initiatives
Intel, a leader in semiconductor manufacturing, is working to integrate its expertise in hardware into the development of quantum computing. Intel’s quantum research focuses on building scalable quantum processors that can be integrated with conventional computing systems. Intel’s Horse Ridge platform, for example, is designed to control quantum bits (qubits) with integrated circuits, enabling more efficient operation of quantum computers.
The company is also exploring silicon-based qubits, which could potentially be easier to scale up compared to other types of qubits. This approach leverages Intel’s expertise in microelectronics, with the goal of making quantum computers more practical for real-world applications.
2. Startups Pushing the Boundaries of Quantum Innovation
While large corporations lead the charge, a number of startups are also making significant strides in quantum computing. These smaller companies are often more agile and innovative, contributing new approaches and solutions that complement the work being done by the industry giants.
a) Rigetti Computing
Rigetti Computing is a California-based startup that has emerged as a major player in the quantum computing space. Rigetti focuses on developing quantum hardware and cloud-based quantum computing platforms. Its Forest platform allows developers to create and run quantum algorithms on quantum processors via the cloud.
Rigetti’s hybrid quantum-classical systems, which combine quantum processors with classical computing power, allow for more practical applications in machine learning, optimization, and cryptography. Rigetti is also exploring new approaches to scaling up quantum processors, making it an important player in the race to build a useful quantum computer.
b) IonQ
IonQ is another promising startup focused on building practical quantum computers. The company uses trapped-ion qubits, which are stable and can be manipulated with great precision, offering the potential for highly accurate quantum computations. IonQ has achieved major milestones, including creating one of the world’s most advanced quantum computers.
IonQ’s approach to quantum computing emphasizes scalability and precision, two critical factors for the practical deployment of quantum systems. The company has also partnered with cloud services like Microsoft Azure and Amazon Braket to provide access to its quantum processors over the cloud, enabling quantum computing for a broader audience.
c) D-Wave Systems
While D-Wave Systems is considered a leader in quantum computing, the company stands apart by focusing on a specific quantum computing approach: quantum annealing. This method is particularly well-suited for optimization problems, making D-Wave’s technology applicable in industries like logistics, finance, and healthcare.
D-Wave’s Advantage quantum computer, released in 2020, boasts over 5,000 qubits and is designed to tackle complex optimization tasks that classical computers struggle to handle. D-Wave is also working on integrating its quantum computers with classical computing systems to provide hybrid solutions.
3. Research Institutions Advancing Quantum Theory
Quantum computing research isn’t just happening in private industry. Leading academic institutions around the world are conducting cutting-edge research into quantum mechanics, quantum algorithms, and quantum hardware. Universities and research labs play a vital role in pushing the theoretical boundaries and providing the foundation for quantum computing’s practical application.
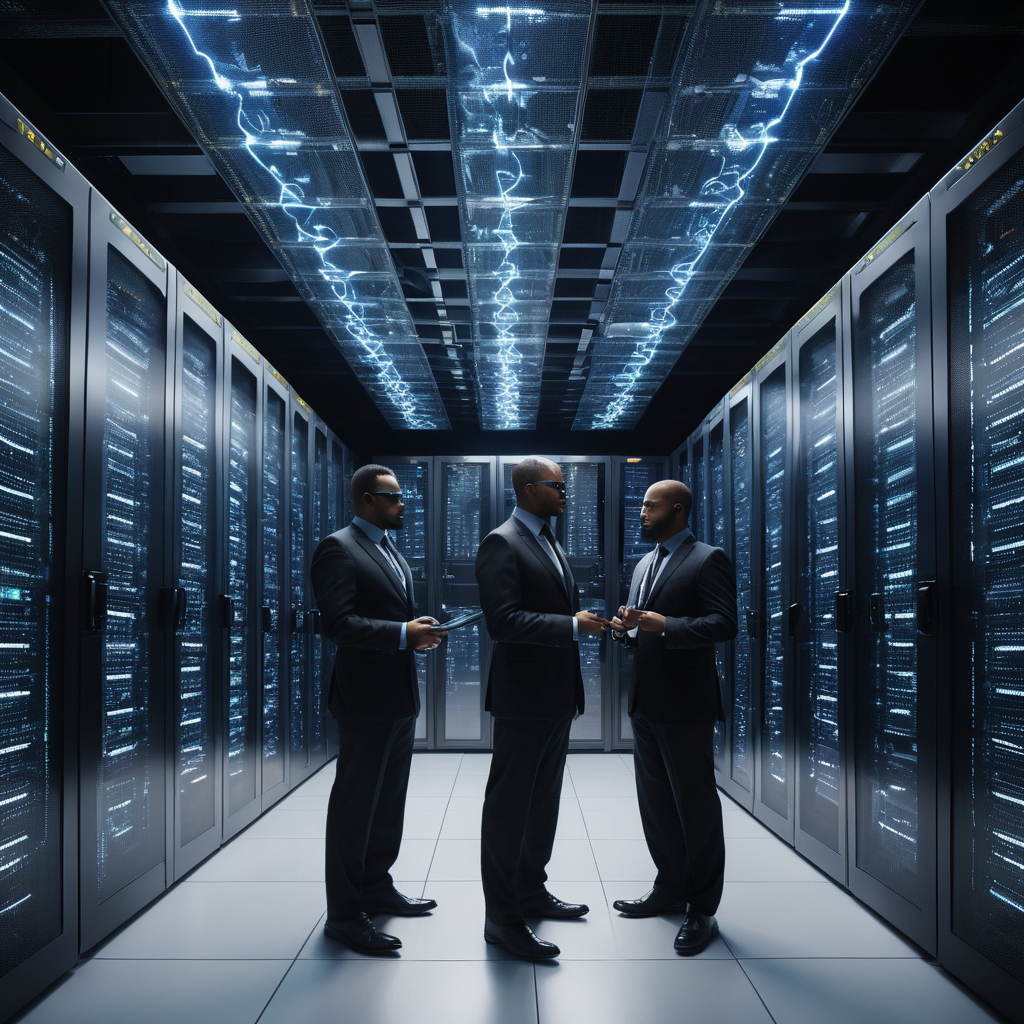
a) Massachusetts Institute of Technology (MIT)
MIT has been a pioneering institution in quantum computing research, with many of its professors and researchers leading the field in quantum mechanics, quantum algorithms, and quantum hardware development. MIT’s Research Laboratory of Electronics and Center for Quantum Engineering are central to its work in quantum computing.
MIT is exploring multiple aspects of quantum computing, from new materials and qubit technologies to quantum error correction and quantum algorithms. The university’s Quantum Information Science program provides an interdisciplinary approach, combining physics, computer science, and electrical engineering.
b) University of California, Berkeley
The University of California, Berkeley is home to some of the world’s top quantum computing researchers. The university’s Berkeley Quantum Computing Center and QISE (Quantum Information Science and Engineering)research program are contributing to advances in quantum algorithms, quantum theory, and quantum hardware.
Researchers at UC Berkeley are exploring quantum machine learning and quantum chemistry, aiming to develop algorithms that will harness the power of quantum computing for solving real-world problems. The university is also collaborating with tech giants and startups to bridge the gap between theoretical research and practical applications of quantum computing.
c) University of Oxford
The University of Oxford in the United Kingdom is another leader in quantum computing research. Oxford’s Department of Physics and Oxford Quantum division are at the forefront of quantum computing and quantum information science. The university is particularly strong in the development of quantum algorithms, quantum cryptography, and quantum simulations.
Oxford is collaborating with several organizations, including Microsoft and IBM, to advance quantum computing research. The university’s interdisciplinary approach brings together physicists, computer scientists, and engineers to develop scalable quantum technologies that could revolutionize fields such as medicine, energy, and communications.
Conclusion: Collaborative Effort Toward Quantum Excellence
Quantum computing’s future will be shaped by the continued collaboration and innovation of large tech companies, nimble startups, and top-tier academic institutions. These key players are contributing to every aspect of quantum computing, from hardware and software development to theoretical research and practical applications. The collective efforts of these stakeholders will determine the pace at which quantum computing transitions from theoretical research to real-world solutions, unlocking transformative potential across industries.
D. Quantum Algorithms and Software Development
As quantum hardware continues to evolve, quantum algorithms and software play a crucial role in unlocking the potential of quantum computing. The development of efficient quantum algorithms is critical for solving complex problems that classical computers cannot handle in a reasonable timeframe. Quantum algorithms take advantage of the principles of quantum mechanics, such as superposition, entanglement, and quantum parallelism, to perform computations that would be intractable for traditional systems. This section delves into the development of quantum algorithms, their applications, and the challenges faced by the quantum software ecosystem.
1. The Basics of Quantum Algorithms
Quantum algorithms are fundamentally different from classical algorithms in how they process and manipulate data. Traditional algorithms rely on binary logic, using bits that represent either a 0 or 1. In contrast, quantum algorithms operate using qubits (quantum bits), which can exist in multiple states simultaneously due to superposition. Furthermore, quantum algorithms exploit entanglement, a phenomenon where qubits become interconnected in such a way that the state of one qubit can depend on the state of another, regardless of the distance between them.
a) Superposition and Parallelism
One of the primary advantages of quantum algorithms is the ability to perform parallel computations. Thanks to superposition, a qubit can represent both 0 and 1 at the same time. This allows quantum computers to explore multiple solutions simultaneously. For instance, in a classical search algorithm, only one solution is tested at a time. However, in a quantum search algorithm, multiple solutions can be tested in parallel, drastically reducing computation time for certain types of problems.
The power of superposition becomes evident in quantum algorithms such as Grover’s Algorithm, which offers a quadratic speedup for unstructured search problems. This could have significant implications for searching large databases or solving optimization problems.
b) Quantum Interference and Measurement
Quantum algorithms also make use of quantum interference to amplify correct solutions and suppress incorrect ones. In a quantum algorithm, qubits are manipulated to interfere in such a way that the probability of measuring the correct solution is maximized. After many rounds of manipulation and interference, a quantum measurement collapses the qubits’ superpositions into a definite state, revealing the answer.
One key challenge in developing quantum algorithms is ensuring that the interference is constructive and that the correct solution is amplified while others are canceled out. This requires sophisticated control over quantum operations, which is an area of active research.
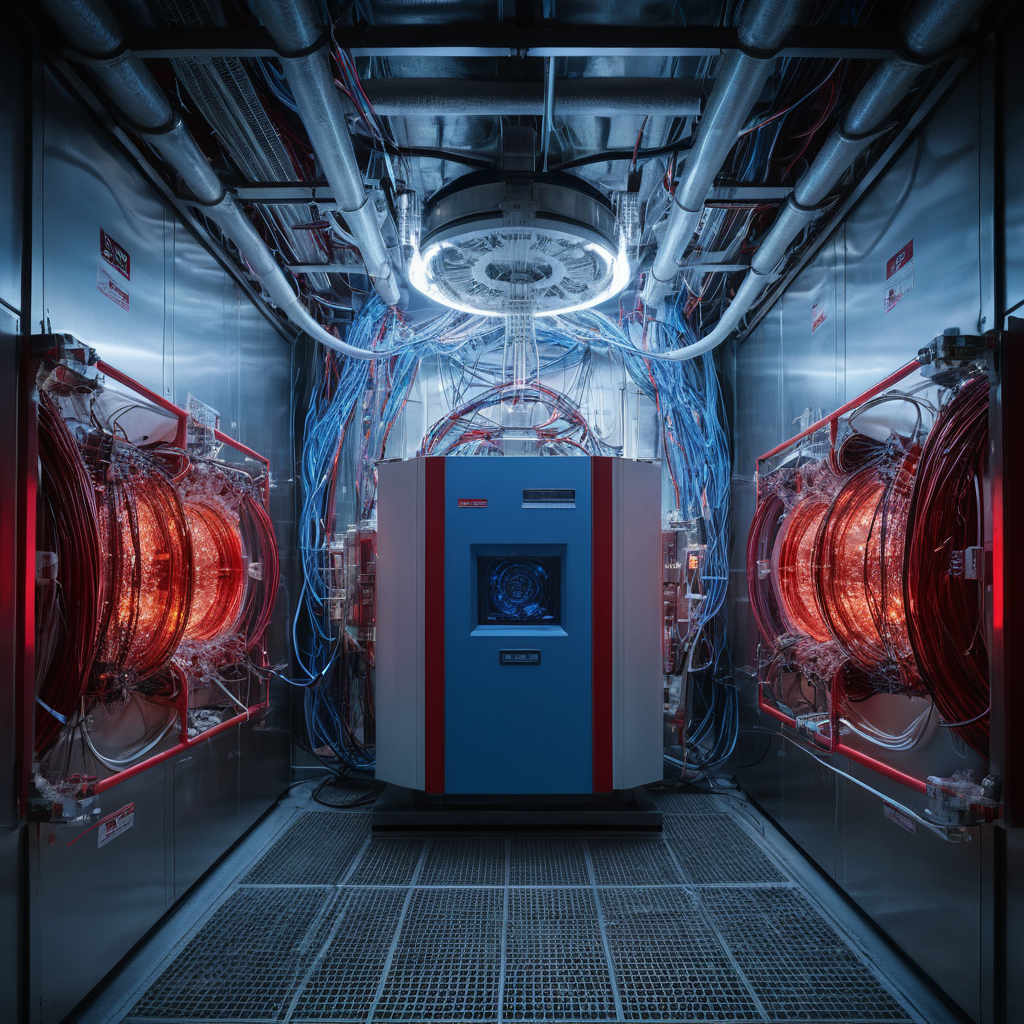
2. Key Quantum Algorithms
Quantum algorithms are expected to revolutionize a wide range of fields, from cryptography to optimization. While the field is still in its infancy, several key quantum algorithms have been developed that demonstrate the potential power of quantum computing.
a) Shor’s Algorithm for Integer Factorization
Shor’s Algorithm, developed by mathematician Peter Shor in 1994, is one of the most famous quantum algorithms. It provides an efficient method for factoring large integers exponentially faster than the best-known classical algorithms. This is a game-changer for cryptography, particularly for public-key cryptosystems like RSA, which rely on the difficulty of factoring large numbers.
In classical computing, factoring large numbers is computationally expensive, and this difficulty underpins the security of many encryption systems. However, Shor’s Algorithm could potentially break these systems, making it necessary for the cryptography community to explore quantum-resistant algorithms to prepare for the advent of practical quantum computers.
b) Grover’s Algorithm for Database Search
Grover’s Algorithm, developed by Lov Grover in 1996, is another key quantum algorithm that offers a quadratic speedup over classical search algorithms. While classical search methods examine each item in a database one at a time, Grover’s Algorithm can search an unsorted database of NN items in approximately O(N)O(N) time, compared to the classical
c) Quantum Approximate Optimization Algorithm (QAOA)
The Quantum Approximate Optimization Algorithm (QAOA) is a hybrid quantum-classical algorithm designed to solve optimization problems. QAOA is aimed at providing approximate solutions to difficult optimization problems, particularly those that arise in fields like machine learning, logistics, and finance.
The algorithm works by using a quantum computer to explore the solution space in a way that is difficult for classical computers to replicate. While the exact solutions may not always be achievable, QAOA provides near-optimal solutions for complex problems. This hybrid approach leverages the strengths of both quantum and classical computing, and it is one of the most promising approaches for near-term quantum computers.
3. Quantum Software Development Frameworks
To make quantum computing accessible and practical, software frameworks and programming languages are being developed to help researchers and developers write quantum algorithms and run them on quantum hardware. These frameworks abstract much of the complexity of quantum computing, enabling users to focus on algorithm design rather than the intricacies of quantum hardware.
a) IBM’s Qiskit
Qiskit, developed by IBM, is one of the most widely used quantum programming frameworks. It provides an open-source platform for quantum computing, offering tools for algorithm development, circuit simulation, and quantum hardware access. Qiskit is designed to be user-friendly, enabling programmers with classical computing backgrounds to quickly learn how to develop quantum algorithms.
Qiskit supports both quantum circuit-based programming and the simulation of quantum systems, allowing developers to test their algorithms on simulated quantum environments before running them on actual quantum hardware. IBM also offers access to real quantum processors through the IBM Quantum Experience, an online platform that enables users to run quantum algorithms on IBM’s quantum hardware.
b) Microsoft’s Q# and Quantum Development Kit
Microsoft’s quantum programming language, Q#, is part of the company’s Quantum Development Kit. Q# is designed to integrate quantum programming with existing software tools, allowing developers to write quantum algorithms while leveraging classical computing systems for parts of the computation. The language provides a high-level abstraction of quantum operations, simplifying the development process.
Microsoft’s Quantum Development Kit also includes simulators and debugging tools that enable developers to test their quantum programs on classical computers. This makes it easier for developers to experiment with quantum algorithms without requiring access to a quantum computer.
c) Google’s Cirq
Cirq, developed by Google, is an open-source quantum software framework specifically designed for Noisy Intermediate-Scale Quantum (NISQ) devices, which are the types of quantum computers available in the near term. Cirq provides tools for designing and simulating quantum circuits and allows users to run quantum programs on Google’s quantum processors, such as Sycamore.
Cirq focuses on optimizing quantum circuits for NISQ devices, which are expected to have limited qubit count and be prone to noise. As quantum computers become more powerful, Cirq is expected to evolve, enabling more complex quantum programs and algorithms to be executed efficiently.
4. Challenges in Quantum Software Development
While quantum software development holds immense promise, several challenges remain in making quantum algorithms scalable and practical for real-world applications.
a) Quantum Error Correction
One of the biggest challenges in quantum software development is quantum error correction. Quantum systems are highly sensitive to external disturbances and noise, making it difficult to maintain the integrity of quantum states during computation. Without error correction, quantum computers are prone to “decoherence,” where the quantum state collapses prematurely, leading to incorrect results.
Researchers are exploring several error correction techniques, such as surface codes and concatenated codes, to ensure that quantum computations remain reliable. However, implementing error correction requires additional qubits, which increases the hardware complexity and poses scalability challenges.
b) Limited Qubit Fidelity
Another challenge is limited qubit fidelity, meaning that the individual qubits used in quantum algorithms may not operate with perfect accuracy. The performance of quantum algorithms depends on how well the qubits can maintain their quantum state and how accurately quantum gates are applied to manipulate those states. Improving qubit fidelity is essential for achieving reliable, large-scale quantum computation.
c) Lack of Quantum-Classical Integration
Quantum algorithms often require classical computation to complement quantum operations. Integrating quantum computing with classical systems in a seamless manner is a significant challenge. Quantum computers can provide exponential speedup for certain problems, but many applications will require hybrid quantum-classical solutions. Developing algorithms that can effectively leverage both quantum and classical systems is a critical area of research.
Conclusion
Quantum algorithms and software development are pivotal to realizing the full potential of quantum computing. With algorithms like Shor’s and Grover’s showing promise in fields such as cryptography and optimization, and frameworks like Qiskit, Q#, and Cirq providing the tools for quantum software development, the future of quantum computing looks incredibly bright. However, challenges like error correction, qubit fidelity, and the integration of quantum-classical systems must be addressed to ensure that quantum computers can meet the needs of real-world applications. As research progresses, quantum algorithms will continue to evolve, unlocking new opportunities in industries ranging from cryptography to artificial intelligence.
E. Quantum Computing and Its Impact on Industries
Quantum computing is poised to revolutionize industries by solving problems that are currently intractable for classical computers. With its ability to perform complex calculations at unprecedented speeds, quantum computing has the potential to transform fields such as pharmaceuticals, logistics, artificial intelligence (AI), materials science, cryptography, and energy. This section explores the potential impact of quantum computing on various industries, how it can enhance efficiency, and the challenges that industries must overcome to leverage this technology effectively.
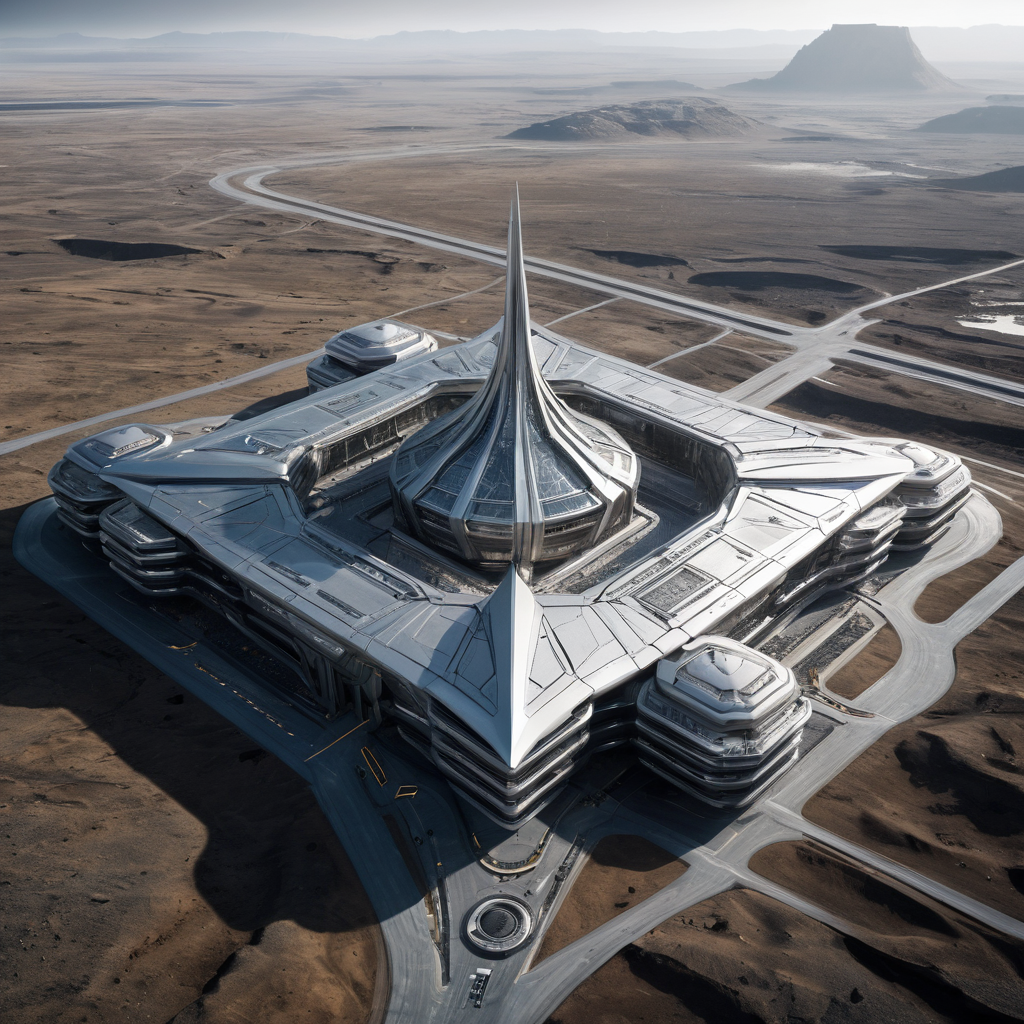
1. Pharmaceuticals and Drug Discovery
The pharmaceutical industry is one of the most promising fields where quantum computing can have a transformative impact. Traditional drug discovery processes involve screening millions of compounds to identify potential candidates for new medicines, a time-consuming and costly procedure. Quantum computers, with their ability to model complex molecular structures and interactions at a quantum level, could significantly accelerate this process, reducing time to market for new drugs.
a) Quantum Simulations for Drug Design
Quantum computing allows for the simulation of molecular interactions at the level of individual atoms and electrons. This means that quantum computers can simulate the behavior of molecules in a much more accurate and detailed way than classical computers, which rely on approximations to model molecular systems. For example, quantum computers can simulate protein folding, a critical aspect of drug design, much more efficiently. This could lead to faster development of drugs for diseases that currently have limited treatment options.
The Quantum Pharmaceutical Program (QPP), an initiative to explore the applications of quantum computing in drug discovery, is an example of how quantum computing can be applied in real-world pharmaceutical research. By using quantum computers to analyze chemical reactions and molecular behavior, pharmaceutical companies can identify promising drug candidates much more rapidly and accurately.
b) Personalized Medicine
Quantum computing can also assist in the development of personalized medicine, which tailors treatment to individual patients based on their genetic makeup. By analyzing large datasets of genetic information, quantum computers could help identify genetic variants that influence disease susceptibility and treatment responses. This would allow for the development of more targeted and effective therapies, potentially improving patient outcomes and reducing the costs of healthcare.
2. Materials Science and Manufacturing
Quantum computing holds significant promise for the materials science and manufacturing sectors, where it can help researchers discover new materials with unique properties. These materials could be used in a variety of applications, such as energy storage, electronics, and manufacturing, leading to more efficient processes and products.
a) Quantum Chemistry for Materials Discovery
Quantum computers are particularly well-suited for simulating the properties of new materials. The ability to calculate electronic structures with high precision could accelerate the discovery of novel materials that could be used in industries such as semiconductors, batteries, and solar cells. Materials with improved electrical conductivity, better energy storage capabilities, or enhanced strength could revolutionize industries ranging from electronics to clean energy.
For instance, researchers are investigating quantum computing’s potential to improve lithium-ion batteries, which are widely used in electric vehicles and consumer electronics. Quantum simulations could lead to the development of batteries that are cheaper, more efficient, and have a longer lifespan, helping to address challenges related to energy storage and sustainability.
b) Advanced Manufacturing Techniques
Quantum computing can also help optimize manufacturing processes. Industries such as aerospace, automotive, and electronics manufacturing rely on precision engineering and complex simulations to design and manufacture high-quality products. Quantum algorithms could be used to optimize production schedules, supply chain logistics, and even the design of new products with better performance.
Moreover, quantum computing could be used to improve additive manufacturing (3D printing) by enabling the creation of highly optimized materials with custom properties. This would allow manufacturers to produce products that are not only stronger but also lighter, more energy-efficient, and tailored to specific needs.
3. Artificial Intelligence and Machine Learning
Artificial Intelligence (AI) and Machine Learning (ML) are already driving innovations across numerous industries, from healthcare to finance. Quantum computing has the potential to supercharge AI and ML by providing faster data processing capabilities and enabling more complex models. This could lead to breakthroughs in pattern recognition, natural language processing, and predictive analytics, among other applications.
a) Quantum-Enhanced Machine Learning
Quantum computing can enhance machine learning algorithms by allowing them to process vast amounts of data more efficiently. Classical machine learning techniques are limited by computational power, especially when dealing with high-dimensional data. Quantum algorithms, on the other hand, can process data in parallel, allowing for faster and more accurate predictions.
Quantum-enhanced machine learning could have significant applications in fields such as autonomous vehicles, fraud detection, and predictive healthcare. For instance, quantum algorithms could enable faster training of neural networks, which are the backbone of many AI systems, making AI more effective in identifying complex patterns and making predictions.
b) Quantum Computing in Natural Language Processing (NLP)
Natural Language Processing (NLP) is another area where quantum computing could have a major impact. NLP involves the ability of machines to understand, interpret, and generate human language. Quantum computing could be used to process and analyze large volumes of textual data more efficiently, enabling the development of more advanced chatbots, virtual assistants, and language translation systems.
By improving the efficiency of NLP tasks, quantum computers could enhance applications in customer service, content creation, and real-time translation, offering more nuanced and contextually aware AI interactions.
4. Cryptography and Cybersecurity
One of the most well-known and debated impacts of quantum computing is its potential effect on cryptography. Modern cryptographic systems, including RSA encryption and Elliptic Curve Cryptography (ECC), rely on the difficulty of factoring large numbers or solving discrete logarithms. Quantum computers, with their ability to perform these calculations exponentially faster than classical computers, could render these cryptographic techniques obsolete.
a) Quantum-Safe Cryptography
In response to this threat, researchers are developing quantum-safe cryptographic algorithms that are resistant to quantum attacks. These new algorithms use mathematical problems that are believed to be hard for both classical and quantum computers to solve. Examples include lattice-based cryptography and hash-based cryptography, which are currently being investigated as potential candidates for post-quantum cryptography.
Governments and organizations are already working to standardize quantum-safe cryptographic methods to ensure that the digital infrastructure remains secure in a post-quantum world. For instance, the National Institute of Standards and Technology (NIST) has launched a project to develop quantum-resistant cryptographic algorithms, which is expected to be completed in the coming years.
b) Quantum Key Distribution (QKD)
Another potential application of quantum computing in cryptography is Quantum Key Distribution (QKD). QKD leverages the principles of quantum mechanics, such as the Heisenberg uncertainty principle, to securely exchange encryption keys. With QKD, any attempt to intercept or eavesdrop on the communication would disturb the quantum state of the system, alerting the parties to the presence of an intruder.
QKD has the potential to provide unconditional security in communications, making it particularly valuable for sectors like banking, military communications, and government networks. Several companies and research institutions are already working to implement QKD in practical systems, with the goal of creating a quantum-safe communications infrastructure.
5. Energy and Sustainability
The energy sector stands to benefit significantly from quantum computing, particularly in the areas of energy production, storage, and distribution. With the global push toward renewable energy and sustainability, quantum computing could help address some of the most pressing challenges in these areas.
a) Optimizing Energy Grid Management
Quantum computing could help optimize the management of smart grids, which are designed to improve the efficiency of electricity distribution. By modeling complex energy distribution networks and forecasting energy demand more accurately, quantum computers could help utilities optimize power generation, reduce energy loss, and improve grid reliability. This would lead to lower costs and a more sustainable energy infrastructure.
b) Renewable Energy and Materials Innovation
Quantum computers can also be used to develop new materials for solar cells, batteries, and wind turbines. By simulating the atomic-level interactions of materials, quantum computing can help discover new materials that are more efficient and cost-effective for renewable energy technologies.
For example, researchers are using quantum computing to design photovoltaic materials for solar panels that can convert sunlight into energy more efficiently. Quantum simulations could also lead to breakthroughs in energy storagetechnologies, such as creating batteries that store more energy for longer periods, essential for supporting the transition to renewable energy.
Conclusion
Quantum computing is set to impact a wide range of industries, from pharmaceuticals to energy, by solving complex problems that classical computers cannot handle. The pharmaceutical industry stands to benefit from faster drug discovery, materials science can benefit from new, high-performance materials, and AI will be enhanced through quantum-powered machine learning. Cryptography and cybersecurity will also face significant transformations, with the need for quantum-safe methods becoming critical. As the technology matures, quantum computing has the potential to drive innovation, improve efficiency, and create more sustainable solutions across these sectors. However, the challenges related to scalability, error correction, and hardware limitations will need to be addressed before quantum computing can reach its full potential in industry.
Notable Books, Research Papers, and Innovations
Artificial Intelligence (AI) and Machine Learning (ML) have spurred groundbreaking advancements in technology, business, healthcare, and beyond. The continuous development of AI has been guided and inspired by key contributions from scholars, researchers, and innovators. This section highlights some of the most influential books, research papers, and innovations that have shaped the AI landscape.
1. Books on Artificial Intelligence
Books have served as a cornerstone for understanding the theoretical and practical aspects of AI. Here are some notable contributions:
a) Artificial Intelligence: A Modern Approach by Stuart Russell and Peter Norvig
This book is considered a seminal text in AI, covering a wide range of topics, from the basics of search algorithms and knowledge representation to advanced concepts like machine learning and robotics. It is widely used in academic and industry circles as a comprehensive guide to the field.
b) Deep Learning by Ian Goodfellow, Yoshua Bengio, and Aaron Courville
This book delves into the principles of deep learning, a subset of AI, and provides an in-depth understanding of neural networks, optimization, and applications in fields like vision and language processing.
c) Superintelligence: Paths, Dangers, Strategies by Nick Bostrom
This book explores the existential risks and ethical dilemmas posed by AI surpassing human intelligence. It provides valuable insights into how AI could shape humanity’s future and stresses the need for responsible development.
d) Prediction Machines: The Simple Economics of Artificial Intelligence by Ajay Agrawal, Joshua Gans, and Avi Goldfarb
Focusing on the economic implications of AI, this book simplifies complex AI concepts and highlights how AI can reduce prediction costs and transform industries.
e) The Master Algorithm: How the Quest for the Ultimate Learning Machine Will Remake Our World by Pedro Domingos
This book offers an accessible explanation of machine learning and discusses the search for a unified algorithm that could address all learning tasks.
2. Landmark Research Papers
Research papers have been instrumental in advancing AI by introducing novel concepts, methodologies, and applications. Some groundbreaking papers include:
a) “A Theory of Communication” by Claude Shannon (1948)
Though not strictly an AI paper, Shannon’s work laid the foundation for information theory, which is fundamental to modern machine learning algorithms.
b) “ImageNet Classification with Deep Convolutional Neural Networks” by Alex Krizhevsky, Ilya Sutskever, and Geoffrey Hinton (2012)
This paper introduced the AlexNet model, which revolutionized computer vision by demonstrating the effectiveness of convolutional neural networks (CNNs) on large datasets.
c) “Attention Is All You Need” by Vaswani et al. (2017)
This paper introduced the Transformer architecture, which has since become a cornerstone of natural language processing (NLP) and generative models like GPT.
d) “Mastering the Game of Go with Deep Neural Networks and Tree Search” by Silver et al. (2016)
This paper showcased AlphaGo, the AI developed by DeepMind that defeated a world champion in the complex game of Go, marking a milestone in reinforcement learning.
e) “Generative Adversarial Networks” by Ian Goodfellow et al. (2014)
This paper introduced GANs, a framework for generating synthetic data, which has applications ranging from image synthesis to drug discovery.
3. Key Innovations in AI and ML
Several innovations have significantly influenced AI’s evolution, demonstrating its capabilities across industries and real-world applications.
a) Neural Networks and Deep Learning
The development of deep neural networks has enabled breakthroughs in image recognition, speech processing, and more. Innovations like ResNet and BERT have become benchmarks in their respective fields.
b) Natural Language Processing (NLP)
AI models like OpenAI’s GPT-3 and Google’s BERT have revolutionized NLP, enabling machines to generate human-like text, translate languages, and understand context.
c) Autonomous Systems
AI-driven autonomous systems, such as self-driving cars and drones, showcase AI’s potential to navigate and make decisions in real-time environments. Companies like Tesla, Waymo, and Amazon are at the forefront of these innovations.
d) AI in Healthcare
AI applications in healthcare include diagnostic tools powered by deep learning, personalized medicine, and robotic surgery. IBM Watson and Google’s DeepMind Health have demonstrated AI’s ability to enhance patient care.
e) Ethical AI Frameworks
Initiatives like Google’s AI Principles and the European Union’s AI Act represent efforts to develop AI responsibly. These frameworks address issues like transparency, bias, and accountability, ensuring ethical deployment.
4. Noteworthy AI Platforms and Tools
The development of sophisticated AI platforms and tools has enabled researchers and developers to experiment, innovate, and deploy AI solutions at scale.
a) TensorFlow
Developed by Google, TensorFlow is an open-source framework widely used for building machine learning and deep learning models.
b) PyTorch
Preferred by many researchers for its flexibility and ease of use, PyTorch is a deep learning framework backed by Meta AI.
c) OpenAI Codex
This AI system powers GitHub Copilot, enabling developers to write code faster and with fewer errors by offering intelligent suggestions.
d) IBM Watson
IBM Watson’s suite of AI tools supports applications in business, healthcare, and education, offering capabilities like language understanding and predictive analytics.
e) AlphaFold
Developed by DeepMind, AlphaFold predicts protein folding structures, a breakthrough in biological sciences with implications for drug discovery and disease understanding.
5. Emerging Innovations
Recent advancements in AI indicate exciting possibilities for the future:
a) AI-Powered Creativity
Generative AI models like DALL-E and Stable Diffusion are pushing the boundaries of creative expression by generating art, music, and literature.
b) Federated Learning
This approach allows AI models to learn collaboratively from decentralized data sources while preserving privacy, showing promise in sectors like healthcare and finance.
c) Quantum-Enhanced AI
Integrating quantum computing with AI has the potential to solve problems that are currently computationally intractable, such as simulating complex systems or optimizing large-scale networks.
d) AI for Climate Change
AI models are being developed to monitor deforestation, optimize renewable energy grids, and model climate change scenarios, showcasing AI’s role in sustainability.
- Books
- Quantum Computing Since Democritus by Scott Aaronson
- Quantum Computation and Quantum Information by Michael A. Nielsen and Isaac L. Chuang
- Quantum Mechanics: The Theoretical Minimum by Leonard Susskind and Art Friedman
- Research Papers
- “Quantum Supremacy Using a Programmable Superconducting Processor” (Nature)
- “The Role of Quantum Computing in Solving Optimization Problems” (Journal of Applied Physics)
- Innovations
- Google’s Sycamore Processor: Achieved quantum supremacy.
- IBM Quantum Experience: Provides cloud-based quantum computing access.
- Quantum Key Distribution Systems by ID Quantique: Advances in quantum cryptography.